- Point of view
Doing more with less in the age of digital transformation
Discover how artificial intelligence (AI) and analytics drive innovation, making organizations more agile and efficient
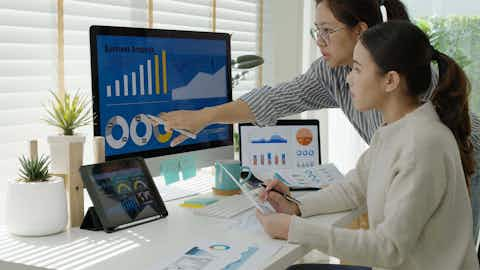
Though enterprise leaders have made strides in advancing their digital agendas, economic headwinds and a rapidly changing business landscape are forcing them to do more with less – fewer people, lower budgets, and minimal costs.
In years past, such a disconnect between available resources and business mandates would have created an impasse. Today, rather than slowing digital transformation, teams can use technology to thrive amid the constraints.
Consider the importance and wide adoption of artificial intelligence technology across industries, which is quickly transforming how we work. Whether it's generative AI like DALL·E 2, using machine learning (ML) algorithms to create images from text, or ChatGPT, an AI chatbot that uses deep learning techniques to produce human-like speech, the potential for business use is endless.
AI, data, and analytics have the potential to help businesses do more, even when they have less. Here, we'll explore how AI supports a data-driven enterprise and the three ingredients for success.
Exploring the power of AI and ML
For most organizations, AI is far from a golden ticket. The rapid transformation it fuels has also brought new challenges. According to a recent study by McKinsey, more than 75% of enterprises have piloted some form of AI, yet less than 15% have realized a meaningful, scalable impact.
Even worse, many leaders often see data governance as a tax instead of a strategic initiative, leading to substandard risk management. As a result, teams often struggle to manage, access, understand, and – most importantly – trust their data.
And these issues only increase when data engineers layer black-box models on top of biased data. This approach leads to unintended consequences such as discrimination, prompting teams to waste time and resources retraining models.
For artificial intelligence and machine learning projects to succeed, it's crucial to have strong data governance and a well-defined framework for responsible innovation, such as machine learning operations (MLOps). By embracing industry best practices to manage complex ML models from the start, teams can operate efficiently, improving the speed, quality, and reliability of artificial intelligence in business.
What's more, through MLOps and a solid data foundation, enterprise leaders can effectively scale and replicate ML solutions across the business for a greater return on investment.
Case study
Enhancing decision-making with AI
After nearly 100 years at the top of the global reinsurance market, a reinsurer was in danger of falling behind the competition. To keep up with digitally transformed insurtech companies, it needed to cut underwriting response time from over a week to under two hours.
And with minimal investments, the company achieved maximum results. We started by optimizing its IT infrastructure and securely transitioning its data to the cloud. With this foundation in place, we connected data from multiple systems into one data pipeline. Then we put this data to use, feeding it into two new ML models we created to empower underwriters to identify the best opportunities rapidly.
By combining the best of human and artificial intelligence, the reinsurer can now produce results for 200 entries in just 14 seconds. With greater accuracy and efficiency, teams can respond to queries faster while improving the customer experience.
Charting the course to success
Digital transformation has been an elusive target for many companies, more of an aspiration than a reality. However, this trend will likely change. We believe emerging technologies will propel companies to realize their vision faster and with fewer resources.
AI, analytics, and other emerging technologies will become the vehicles of digital transformation, data will become the fuel, and the alignment of people, processes, and partnerships will become the compass.
Let's explore each point of the compass in more detail:
1. People
Thanks to a tight labor market, attracting and retaining talent has become a core challenge for many firms. Large employers have announced significant investments in workforce education and development programs to decrease churn and fill talent gaps.
At Genpact, for example, we launched DataBridge, an initiative to increase data literacy across our organization and shift employees away from transactional projects and into insight-generating roles. In essence, rather than focusing solely on hiring new talent, which is a costly process, we empower 100,000 existing employees with the data analytics skills, tools, and techniques they need to drive value for our customers.
This approach improves retention, too. According to a recent report on talent equity, more than 80% of employees make a switch due to a lack of opportunities. So, upskilling and reskilling employees should sit at the top of every business agenda.
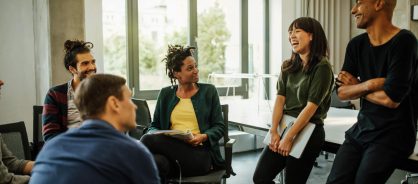
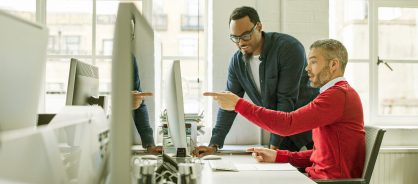
2. Processes
Finding the right talent isn't the only challenge. Enterprise leaders must also review and improve their operating models and processes to make the most of their people. Siloed teams and rigid company structures should become a thing of the past.
Operating models like flow-to-work, in which employers assign teams with unique skills to the highest-priority tasks, help create pools of talent resources that companies can deploy on demand. This talent mobility allows enterprises to simplify processes, incubate pilots, and pivot focus to high-priority projects more effectively. And this benefits employees, too, as they break organizational boundaries, learn new skills, and help to foster a culture of continuous learning.
3. Partnerships
Reaping the benefits of artificial intelligence and other technologies can still prove challenging, even after upskilling employees and refining processes. To go from the 75% of companies that pilot AI programs without meaningful impact to the 15% that succeed, companies must stop trying to make the journey alone.
Historically, business leaders assumed hiring a couple of data scientists would be enough to reap the benefits of AI and ML. However, as technology disruption picks up steam, organizations recognize the need for diverse talent with data science, process, industry, and people management skills. Of course, assembling these teams from the ground up comes at a high cost – hence the value in forming partnerships with technology and data analytics services providers.
Whether powering data-driven decisions with cloud, bolstering business resilience with analytics, driving sustainability with data, building ethical AI, or even protecting intellectual property, technology service providers have an army of experts at their disposal.
To be sure, these partnerships don't replace in-house teams. Instead, they enhance them by filling talent gaps and through knowledge sharing. In short, AI and ML partners with functional business and industry experience can quickly move projects from pilot to production.
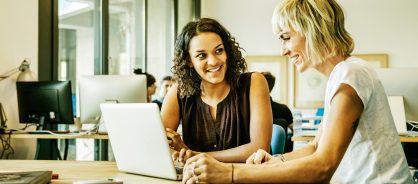
Case study
Driving $300 million in savings with AI
With outdated inventory management and forecasting processes, a global aircraft engine manufacturer struggled to deliver replacement service parts on time. For its clients, delayed parts meant delayed flights – a shortcut only to customer dissatisfaction.
So, we jumped into action. First, to expedite operations, we partnered with the manufacturer's team to digitize the supply chain, using machine learning techniques to analyze demand patterns and anticipate the most common requests. Then, we introduced an automated order fulfillment solution. Finally, we helped the manufacturer identify problems before they happened with an internet of things (IoT) solution for predictive maintenance.
In the first year, the manufacturer saved over $300 million from new planning and supply chain efficiencies and additional savings of $250 million each year from predictive maintenance powered by AI and advanced analytics.
The way forward
The challenge of the past few years was scaling for hyper-growth. And at times, leaders had seemingly unlimited resources to make it happen. Looking ahead, the mandate to use technology to scale insights across the enterprise and spur innovation remains, yet the unlimited resources have dwindled.
Connecting people and processes with the right AI and ML partnerships will educate, equip, and empower employees to make critical decisions using data. In addition, this approach elevates teams from technical and non-technical backgrounds to high levels of data literacy and analytical competency.
With a new operating model, enterprises can tap into their treasure trove of data to spark innovation, manage resources efficiently, and deliver business impact, without delaying their digital transformation goals.