- Point of view
The AI insurance revolution in the era of climate change
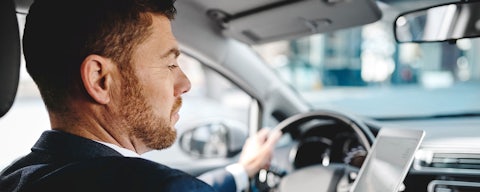
Artificial intelligence (AI) is an invisible force among us. It's already in use across many different areas of our everyday lives, from healthcare to customer service to even the stock market. In the insurance world, its impact will likely be similarly widespread, ranging from automating underwriting and claims to improving customer service and damage assessment. More importantly, it has the potential to combat one of the most pressing issues of our time: climate change. As outlined in our Insurance in the Age of Instinct report, the insurer of the future will harness AI and adopt a whole-system planning approach to respond to the climate emergency and reinforce the industry against this systemic threat.
The increasing threat of climate change
The number of natural disasters that are increasingly linked to climate change and wreaking global havoc is growing. In 2019, natural disasters caused catastrophic damage, from the Australian and Amazonian wildfires to severe flooding in the US and droughts in India. According to Swiss Re, these natural disasters resulted in $133 billion in global economic losses that year[1]. Flooding alone caused an estimated $82 billion in damages in 2019, only $13 billion of which was insured, according to insurance firm Aon[2].
The cost of these catastrophes for both the environment and society is high. This is further compounded by the fact that many of the financial losses caused by these disasters are uninsured, leaving customers, businesses, and governments to pick up the bill.
The widening protection gap
The mounting losses from natural and man-made disasters are increasing the costs of some types of coverage around the world as insurers tighten rates in affected areas. Insurance in hurricane-prone Florida is already much more expensive than it is in northern Europe, for example.
As insurance becomes unaffordable for low- and average-income customers, the protection gap – the difference between total economic loss and the value of assets covered by insurance policies – widens, disproportionately affecting those on the front line of climate change. In the United States, the number of people living in the Midwestern flood plains who have flooding insurance has fallen by 15% in the past decade.
This protection gap is a huge problem for society – data shows that if a larger proportion of the population is insured at the time of a disaster, recovery is quicker and the costs to the taxpayer are lower.
But this protection gap problem is growing. ClimateWise, a global network of 29 insurance industry organizations based at the University of Cambridge, warns that the gap of uninsured or under-insured assets has quadrupled over the past 30 years, posing considerable risk to society as a whole.
AI to the rescue
AI will likely be a promising ally in the insurance industry's efforts to tackle climate change and narrow the protection gap. The technology has the potential to offer benefits to all parties involved, from an easier and quicker claims process for customers to insurers predicting and preventing claims – protecting customers, the economy, and their balance sheets.
And for those who are uninsured or under-insured, the reduced transaction costs of automated technology mean that AI has the power to make insurance more affordable and easier to use.
AI technology is already proving revolutionary in the fight against climate change. Japan is using AI to analyze satellite images of the earth to predict future natural disasters, while NASA and data analysis firm Development Seed recently tracked the path of Hurricane Harvey in the United States six times more accurately than traditional monitoring, helping authorities to prepare in advance.
In the future, insurance companies have an opportunity to become eternal protectors, playing an increasingly proactive role in helping communities better prepare for climate change. These will range from using data to help nudge societies away from poor planning decisions such as overbuilding in high-risk coastal flood zones to encouraging authorities toward better choices such as building more resilient infrastructure designed to mitigate rising sea levels.
AI has the power to significantly reduce headaches for customers who are victims of climate-change-related disasters by tracking and warning customers before an incident occurs. This is something insurance company Hippo already offers. The firm uses smart home sensors and internet of things (IoT) technology to identify and predict damage before it occurs, mitigating the impact of events. After an event, its AI technology enables faster, more accurate, and more seamless claims processing, allowing customers to begin the recovery process more quickly.
AI weather predictions
AI is already making headway in tackling climate change-related disasters. In the UK, the government has recently announced a £1.2 billion investment in the world's most powerful weather and climate supercomputer. The computer will be able to tackle a number of climate-change-related tasks, including more accurately predicting storms and changes to the global climate and selecting optimal locations for flood defenses.
In the US, several major insurance companies are already using an AI-enabled network of rooftop sensors developed by weather tech startup Understory to track and monitor weather patterns for businesses. According to a recent report by IBM, one of these insurers estimates that the technology has already saved it as much as 20% in claims by improving claim accuracy after recent storms.
The data challenge
But AI alone is not a silver bullet that will solve the climate challenge. The quality of its models and decisions will only be as good as the data that feeds into it. AI platform One Concern is a case in point. It recently hit the headlines for allegedly covering up inaccuracies in its data capture; an investigation by the New York Times showed that the company was using inaccurate or incomplete data, such as earthquake simulation maps that were missing data from commercial areas.
Moreover, AI systems that use historical data to predict climate change can often be inaccurate as climate change becomes an ever-evolving and changing threat. Insurers, governments, and businesses must forge strong partnerships and share data and AI resources to ensure that their predictions are as accurate as possible and that they share their learnings across society.
A future collaborative ecosystem
In the face of the growing climate emergency, the insurance industry is facing a moment of reckoning. As Eric Stockwell, head of insurance consulting and transformation services at Genpact states: "Insurance companies must work out how to take advantage of artificial intelligence but also understand their place in a larger ecosystem in order to make it a protective rather than a reactive environment."
Given the scale of the challenge, many are calling for cross-sector collaboration: the insurance firm Aon, for example, recently brought together leaders from the private and public sectors to discuss how they could collectively work together to close the protection gap in a world where climate change disasters are likely to become even more frequent.
The insurance industry will need to join a wider connected ecosystem – comprising not only partnerships between those in the industry, but also governments and non-governmental organizations – and work with them to tackle one of the biggest challenges of our lifetime. Initiatives such as open-source modeling platforms and collaborative ecosystem infrastructures could help to build a system of risk management that will enable society to move away from poor planning and toward vastly improved infrastructures, all fueled by intelligent data. This ecosystem will need to prepare to effectively harness the power of AI to lessen the impact of climate change on all of our lives.