- Point of view
Unlocking the power of machine learning operations for seamless AI integration
Looking to the future of digital transformation
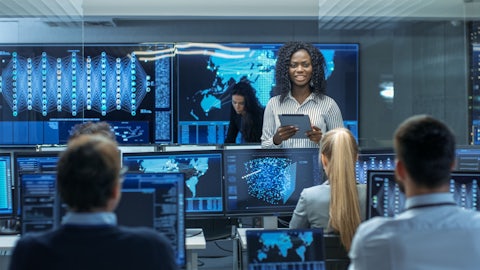
Machine learning (ML) is the backbone of artificial intelligence (AI) development, empowering systems to learn from data, adapt to changing environments, and make informed predictions. Yet, most organizations struggle to capture the full benefits of ML and AI because of inadequate processes.
This lack of structure often results in suboptimal model performance, inefficient business data management, and other missed opportunities. That's why machine learning operations, or MLOps, is crucial in providing a framework for scalable and efficient AI implementation across the business.
With ML operations at the heart of AI development, organizations can quickly turn data into insight and insight into action – the type of decisions that keep customers and employees coming back for more.
It might not be a surprise that AI and ML are among the leading technology investments for Global 2000 enterprises, according to HFS.¹ But it's not enough to simply prioritize AI and ML – the way business leaders approach its use needs to change too.
From experimentation to ML operationalization
Business applications driven by AI models support faster and more intelligent decision-making. However, from our experience working with enterprises across industries, we've witnessed that roughly only half of all AI proofs of concept ever make it to production.
With the swift evolution of AI applications like generative AI, enterprise leaders are under mounting pressure to expedite AI projects and align AI investments with business value. Many organizations also focus on IT modernization to fully exploit AI capabilities.
In short, there has been a shift in how senior executives approach artificial intelligence in the enterprise (figure 1). Today, AI innovation needs to move beyond experimentation to operationalization – fast.
Figure 1. Evolving expectations from enterprise AI initiatives
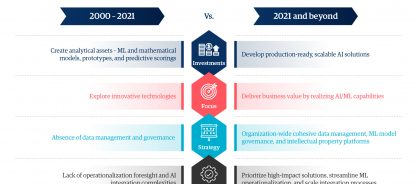
A new approach to AI development is the only way to demonstrate significant top-line and bottom-line benefits connected to better customer experiences, competitive advantage, and business growth.
Exploring common challenges
ML models that go into production must handle large volumes of data, often in real time. Unlike traditional technologies, generative AI and ML technologies deal with probabilistic outcomes – in other words, finding the most likely or unlikely result.
Therefore, the moving parts of the ML model development need close monitoring and quick action when deployed to ensure accuracy, performance, and user satisfaction. Data scientists must grapple with three key influencing factors to ensure the proper development of ML models:
- Data quality: In production environments, the quality, completeness, and semantics of data are critical because ML models rely on data from various sources in different volumes and formats
- Model decay: As the business environment evolves, data patterns in ML models change. This transformation reduces prediction accuracy for models trained and validated on outdated data. Such degradation of predictive model performance is known as concept drift, which makes creating, testing, and deploying ML models challenging
- Data locality: Employing data locality and optimizing access patterns enhances the performance of a specific algorithm. Nonetheless, these ML models may not function accurately in a production setting due to disparities in quality metrics
These factors push ML practitioners to adopt a change-anything, change-everything approach – but these decisions often lead to more problems.
As a result, data scientists waste time and effort navigating technology and infrastructure complexities. Costs increase due to communication and collaboration issues between engineering and data science teams. And because of the trade-off between achieving business goals and providing stable and resilient infrastructure platforms, projects slow. On average, it could take three months to a year to deploy ML models due to changing business objectives that require adjustments to the entire ML pipeline.
Finding a solution
The machine learning life cycle needs to evolve to tackle these challenges. Data and analytics leaders must look for repeatable and scalable data science development processes. In other words, enterprise leaders must rely on machine learning operations.
MLOps help organizations achieve automated and reliable ML model deployment, consistent model training, model monitoring, rapid experimentation, reproducible models, and accelerated model deployment (figure 2). Enterprise leaders can only achieve these benefits through continuous and automated integration, delivery, and training.
Figure 2. MLOps best practices
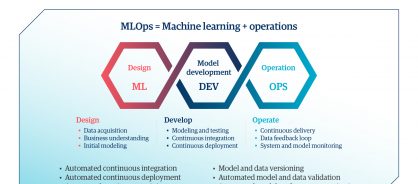
Exploring the benefits
The implementation of MLOps in the enterprise aligns business and technology strategies, delivering multiple benefits such as:
- Rapid innovation: Faster, effective collaboration among teams and accelerated model development and deployment lead to rapid innovation, enabling speed to market
- Consistent results: Repeatable workflows and ML models support resilient and consistent AI solutions across the enterprise
- Increased compliance and data privacy: Effective management of the entire ML life cycle, data, and model lineage optimizes spending on data privacy and compliance regulations
- High return on investment: Efficiently managing ML systems and monitoring model metrics ensures a high return on investment by prioritizing viable use cases and preventing implementation failures
- Enhanced decision-making: IT and data asset tracking, coupled with improved process quality, creates a data-driven culture where employees make decisions based on facts
Bringing MLOps to life in healthcare
A healthcare equipment manufacturer struggled with delays in invoice payments and lacked a solid accounts receivable process. Genpact used MLOps to design a streamlined data-processing and ML model pipeline using open-source tools for rapid integration into existing infrastructure. The solution used 3.6 million invoice metrics for preprocessing and subsequent ML model training. As a result, we developed a fully automated pipeline with daily data feeds to reduce overdue invoices to less than 12% from 20% to 25%.
Getting started
Every organization can reap the benefits of MLOps, particularly as AI adoption continues to grow – more than doubling since 2017, according to McKinsey's research.2 So, how do you develop MLOps in your enterprise? It starts with five steps (figure 3):
- Data engineering: Ensures the foundational data used for machine learning is of high quality and relevance, crucial for accurate and meaningful model outcomes
- Algorithm development: Creating and refining mathematical models is essential for machines to learn patterns and make accurate predictions
- Model deployment: Integrating ML solutions into a production environment enables AI models to make real-time predictions and continuously learn from user interactions
- Model monitoring: Continuous oversight of machine learning solutions increases accuracy and reliability, resulting in the delivery of consistent and trustworthy results
- Model hypercare: Postdeployment support and fine-tuning address any issues, maintaining the ongoing effectiveness and performance of AI models
To be sure, several factors, such as evolving regulatory requirements, unstandardized data collection, and unstable legacy systems, can impact AI model performance. These challenges often result in frequent redeployments in live production environments, which are inefficient and disruptive. On the other hand, effective implementation of MLOps from the start of each project streamlines the entire ML model life cycle, allowing you to pivot and adapt seamlessly.
Figure 3. MLOps as an integral part of the ML model life cycle
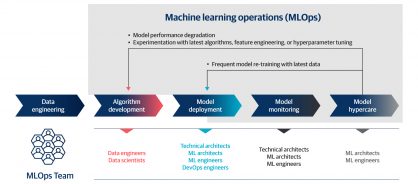
The way forward
By incorporating machine learning operations from the earliest design phases of any AI project, enterprise leaders can harness the full potential of ML – without the headaches. These ML solutions can then be effectively scaled and replicated across the enterprise for greater return on investment and competitive advantage.
Sources
1. "H1 HFS Pulse Survey," HFS Research, 2021.
2. "The state of AI in 2022—and a half decade in review," McKinsey & Company, December 6, 2022.