- Blog
Trust and safety in the era of generative AI
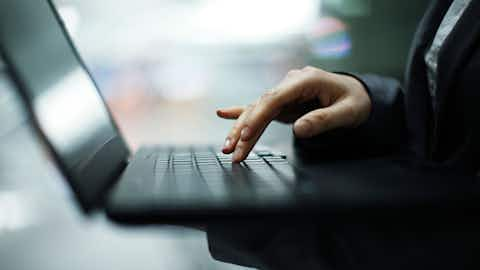
Conversations about the impact of generative AI (gen AI) are everywhere. Online, in classrooms, and in boardrooms. It presents a wealth of opportunities but requires a complete rethink of how businesses operate. For trust and safety, this includes:
- Re-engineering moderation and review processes
- Reassessing safety-by-design product and AI models
- Rethinking content creation and consumption dynamics
And this all needs to happen swiftly and with agility.
Over a series of blogs, I'll explore gen AI's implications for trust and safety. I'll look at:
- The impact of gen AI across different groups and the challenges already surfacing
- How we can proactively address the evolving needs of safety systems and processes
- How Genpact is evaluating and building strategies and capabilities
Let's start with the definition: gen AI can produce original content in response to a user's queries or prompts. By using complex algorithms powered by large data sets and machine learning, AI tools can create human-like prose, digital art, audio, video, computer code, and much more.
These capabilities open new dimensions of possibilities and use cases for AI across all industries. But with these opportunities comes responsibility - we must account for and solve challenges around safety, bias, and the impact to humanity with ethical AI practices.
We can broadly classify the implications of gen AI for trust and safety into three groups:
- Gen AI developers. These are companies building their own models of gen AI, most notably OpenAI (ChatGPT, DaLL.E, Codex), Google (LaMDA), and Meta
- Gen AI integrators. These are companies and their platforms that have integrated gen AI models or adapted gen AI models to suit use cases and functionality on their platforms. These include Bing's integration of OpenAI's gen AI models, Bard's integration of LaMDA, and GitHub's integration of Codex into GitHub Copilot
- Gen AI content amplifiers. These are companies and their platforms on which gen AI-produced information is hosted, shared, or propagated. These platforms are for person-to-person information sharing, the most significant ones being social media sites, content-sharing platforms, and messaging apps. When AI-generated information is introduced and not distinguished, it creates challenges
The biggest learning for trust and safety over the last decade has been to ensure solutions are not an afterthought - they should be central to product design and have integrated safety processes. So, it's critical that gen AI solutions built by trust and safety service providers like Genpact are integrated by design and customized to meet the unique challenges of each industry. This practical approach addresses the nuances of trust and safety considerations and allows teams to develop, implement, and launch agile, innovative solutions.
Gen AI has already surfaced multiple challenges in its nascent stage. The most notable include:
- Quality of training/learning data. Gen AI requires large datasets to meet the needs of varied users. For supervised, unsupervised, and semi-supervised learning models of deep learning, this requires data curation, labeling, and quality controls across a wide array of datasets on different subjects
- Prompt testing and design. When prompted with queries about harmful topics, such as methods for cyberattacks, gen AI has the potential to generate harmful outputs. This requires both automated and manual tests and creative testing of edge cases so data scientists can address and curtail these outputs
- Guardrails. Along with prompt testing, guardrails need to be designed for both inputs and outputs on AI platforms to ensure they aren't generating harmful information when prompted
- Hallucinations. Gen AI models sometimes provide confident but inaccurate, misleading, or harmful outputs. This happens due to inadequate input datasets
- Bias. If the training or learning data is biased, all outputs from gen AI will reflect the same bias because it's modeling outputs from the same dataset. Checking for bias is crucial in the early stages of using gen AI
- Developer-creator integration compliance. Numerous creators and developers use gen AI models to build apps, APIs, and products. There is a significant push and pull of information during this transaction, and robust compliance frameworks and checks are necessary to ensure safety compliance
- Plagiarism and fair use. Trust in gen AI models will erode if the right input and output guardrails aren't built for copyright/intellectual property and fair use
- Regulatory readiness. Regulation of gen AI is a step behind, as in other cases of tech regulation, but building agile models to accommodate regulatory compliance is critical for gen AI
These are a few examples of the challenges that we are working on solutions and building capabilities for. In my next blog, I'll discuss our work on industry-specific challenges and how we solve them.