- Blog
Five ways to make data consumable
In the digital age, getting insights from data shouldn't get complicated
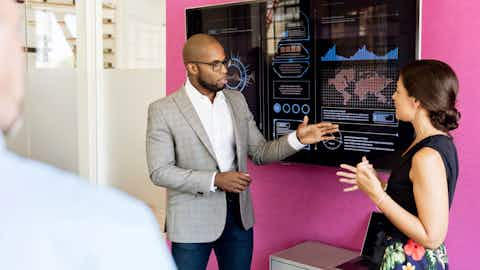
The journey from data to decisions has shortened. Decision makers increasingly demand the right data at the right time and in a consumable format. Yet most organizations are still stuck in data silos. And insights from spreadsheets and other archaic tools don't always add up. This disconnect is making decision makers nervous.
As a result, organizations strive to make their data more consumable and actionable. Welcome to the age of modern data solutions. Across industries, enterprise leaders are digitally integrating and managing big data, improving operational and analytical processes.
The result? Employees and consumers rely on data to guide every decision.
But building modern data solutions is not an easy task. For the organizations ready to act, we've identified three critical elements to make data more usable:
- Data must become accessible. Customers and employees must pull the data they need, when they need it, and on the right device
- Data must become trusted. Decision makers must understand the reliability, robustness, and freshness of their data
- The end-user experience must become paramount. That means starting with the experience in mind and then working backward
A framework for building consumable data solutions
So, how can you make your data more approachable? Here are five ideas.
- Begin with experience. An excellent experience leads to continuous consumption. Consider your end users' needs, learning patterns, and data literacy. How will they consume their data, on what devices, and to what end? Businesses must partner with cloud and analytical providers to deliver seamless experiences every time.
- Improve the trust quotient of your data. When democratizing access to data, end users need to trust, understand, and assess it. When they have confidence in the health of the underlying data, they will rely on data to guide their decisions. Building trust in your data takes time. But it is worth the effort.
- Don't map apples to oranges. Enterprises often store data in functional silos. That leaves many organizations running in circles trying to connect their data across the enterprise. Thankfully, decision makers are tapping into new concepts like the data mesh architecture, which quickly and effectively ingests and manages big data without forcing everything into a data lake first.
- Be hawk-eyed on governance. Ensuring your data is reliable and accessible is only half the battle. Enterprises must audit effectively to build a strong foundation for ethics and governance. Therefore, a framework for sustainable innovation is critical, particularly when using artificial intelligence (AI) and machine learning technologies. By connecting diverse groups of governance custodians, for example, enterprise leaders can catch and remedy unintended AI biases upfront.
- Work smarter, not harder. This stage is where your existing tech stack's maturity starts influencing your ability to evolve. Digital native organizations already have flexible and scalable data architectures on the cloud – the challenge is connecting your technology capabilities and integrating them to deliver meaningful experiences. However, those further behind on their digital journey may need a more comprehensive data strategy to improve data consumption.
Bringing strategy to life
One of the largest distributors of fresh produce in the US struggled to connect its data across its network of independent growers. Plus, the company realized that keeping up with changes in demand was no easy task.
To figure out the root cause of the problem, we partnered with the company's team to analyze how end users, from suppliers to employees, managed and consumed data. We quickly realized that the lack of quality and real-time data resulted in delayed communication of harvest orders between harvest planners and growers. Additionally, immediate visibility into production, demand, and supply-chain changes was nonexistent.
So, we jumped into action, migrating all their data to the cloud and developing applications for real-time updates on harvest orders and forecasting. By building a connected data ecosystem, we reduced peripheral costs by 30%, improved operational efficiencies by 20%, and increased time to market by 50%. More importantly, we significantly enhanced customer and employee satisfaction by making the data consumable and actionable.
Tapping into the treasure trove of data at your fingertips
You can keep modernizing your enterprise landscape, but it will not help you deliver the experience your customers and employees want. As enterprise leaders, we must first understand and correct bad habits and acknowledge that data consumption problems boil down to awareness, literacy, and accessibility.
For starters, bake business context into your data and tailor data offerings for your end consumers. Focus on democratizing access to data across the enterprise and always keep looking for answers to these two questions: Is your data fit for purpose? And did you overcome the friction of getting to it? If the answers to both are yes, you've made your data consumable.