- Blog
Retail media networks are booming. Here's how they can do even better
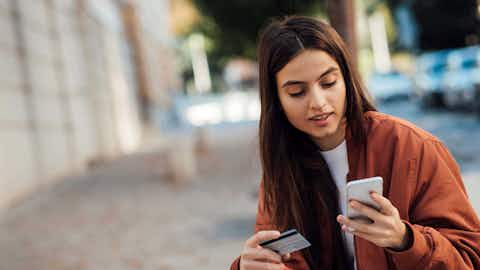
Data sharing between retail and CPG companies for success in RMNs
There's been a lot of buzz around retail media networks (RMNs) over the past few years, but is the hype justified, and will it last? We believe the answer is yes, but only with the right data sharing and analytics in place between retailers and consumer packaged goods (CPG) manufacturers.
RMNs enable CPG companies to buy advertising space across retailers' digital channels, therefore allowing enhanced consumer targeting close to point of purchase.
Their popularity is unsurprising when you look at evidence of their effectiveness. Studies suggest a strong correlation between online product ads and purchase likelihood.
Now the question is: How can retail and CPG companies take full advantage of the growth opportunities that these platforms present?
Collaboration is crucial to RMNs' ongoing success
Advertising and consumer data is understandably closely guarded by retail and CPG companies for reasons such as privacy and standardization to competitive concerns. However, to increase the effectiveness and efficiency of RMNs and reap a high return on investment, it is vital that retailer and CPG manufacturers establish a framework for data sharing.
As a relatively new concept, these networks are full of potential, but as with all fresh ideas, scalability and momentum are key to ongoing success. With the right data infrastructure and analytics capabilities in place, we know RMNs will continue to flourish. They'll do this through enhanced data sharing, better targeting and analytics, and collaborating on relevant and engaging campaigns.
Room for improvement
We've identified three key areas that we believe will support the success of RMNs and capture more growth for retail and CPG companies:
1. Enhanced data sharing
There are certainly challenges to overcome to improve collaboration between retailers and CPG manufacturers, especially when it comes to sharing valuable consumer insights. However, the wealth of first-party data between them has the potential to significantly optimize campaign performance and ROI of RMNs to deliver business value. The CPG and retail partners that do this well will have:
- Clear data-sharing goals linked to business plans
- An agreement on the type of data needed to support those goals to enable growth
- A data governance framework with defined parameters such as ways to constantly monitor and measure the effectiveness of the RMN program
- Advanced analytics and quality data to drive the best insights
- A contractual agreement to establish rules around sharing data
2. Better targeting and analytics
RMNs, by their very nature, offer targeting and analytics capabilities, but they could be even more sophisticated. By sharing data, retail and CPG companies can uncover more insights about target audiences than each party could have achieved alone, such as purchasing behavior, conversion rates, and customer lifetime value. And you can improve targeting efficiency by analyzing which types of buyers interact with your ads.
3. More engaging ads
Consumers are increasingly adept at ignoring all brands' best efforts when it comes to advertising. That's why CPG firms must continually innovate their advertising strategies and formats.
Through collaboration, retail and CPG companies can take full advantage of interactive ad formats offered by RMNs – such as user-generated content, gamification, and personalized ads with targeted offers – to improve customer experiences. Especially with location-based targeting, CPGs can appeal to specific shopper segments.
With the right data and tools, both parties can optimize campaign performance and increase basket sizes.
A win-win future is in sight
RMNs will continue to get more sophisticated. Targeting capabilities will get even better, making the customer experience more seamless. But to take full advantage of these opportunities, retailers and CPG manufacturers must prioritize collaboration and data flow between their organizations. Only then can they achieve a win-win situation where both parties can enjoy profit increases and overall business growth.