- Blog
Three ways enterprise analytics will evolve in 2022
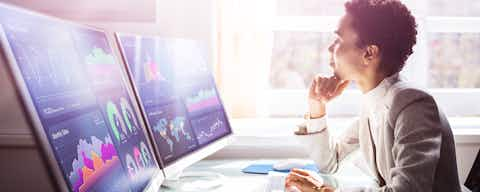
Enterprises of all sizes are embracing new analytics initiatives at an unprecedented pace, transforming how they interact with customers, employees, and other business partners. And I believe this is just the beginning.
In 2022, the speed of digital innovation will only accelerate. As a result, enterprise leaders will need to take on new roles and responsibilities as the demand for data and analytics continues to grow.
In a previous blog, I explored the enterprise analytics trends to look out for next year. Now, I share my predictions on the themes that will drive the evolution of business analytics.
1. Data and analytics leaders move into the spotlight
Enterprise leaders have historically treated analytics as a back-office function. However, the massive demand for data-driven insights has placed the discipline much closer to the front office, especially as data becomes a central decision-making component. With that increased visibility also comes more pressure to democratize access to data across the organization. Subsequently, the role of analytics leaders will expand.
Organizations are making considerable investments in data management, from infrastructure to advanced analytics. But tech is only part of the answer. Even the best-in-class data solutions will fail if they are not helping organizations drive meaningful results.
In practical terms, this means the future of data analytics requires enterprise leaders to put more effort into understanding the business context and industry nuances before applying technologies such as artificial intelligence (AI) or machine learning.
This ambition will increase interactions between departments, making extremely valuable analysts and data scientists who are equally comfortable speaking the languages of business and data. Furthermore, that bilingual ability will become more critical as data analytics expands beyond IT departments into other parts of the enterprise.
2. Analytics becomes a crucial skill for everyone
Think back to the video game experience of 30 years ago compared to today. Over the years, developers have unleashed a massive amount of innovation at both the back end and front end.
Now compare that to the evolution of data analytics. We've seen vast amounts of innovation at the back end with the development of cloud data ecosystems, but little at the front end. The data experience for most businesspeople comes through PowerPoint. They only see the output of tech and analytics teams working behind the scenes.
But years ago, anyone could crunch the numbers themselves using spreadsheets – a familiar tool they could readily access. Now, back-end tech has become sophisticated and complex. Consequently, fewer people in the business can access and produce insights, which is a considerable problem when analytics is in high demand.
Enterprise leaders can address this challenge by upskilling their workforce in data science techniques. By democratizing data across the organization, every employee can tap into the treasure trove of data at their fingertips, producing insights that show management how to lead a digital transformation at scale.
3. Responsible AI becomes a priority
Nearly every industry is adopting AI and machine learning. But ethical questions arise as technology becomes increasingly pervasive behind the scenes in our everyday lives.
The challenge of AI implementations at scale? At the outset, it works based on the available data and human understanding. But as new data enters the equation, the models change. If left unchecked, they can create unintended consequences – some obvious and others not yet known.
Take dating apps as an example. Millions of people use online dating apps powered by predictive models, which users train with each swipe left or right.
But at what point do humans oversee the self-learning algorithms powering decision-making processes? And when should regulators intervene to prevent potential biases or other consequences from the misuse of AI? Essentially, we need controls and measures to manage technology effectively, a framework for responsible AI.
Enterprise leaders can address this challenge by upskilling their workforce in data science techniques. By democratizing data across the organization, every employee can tap into the treasure trove of data at their fingertips, producing insights that show management how to lead a digital transformation at scale.
Leading the way in digital transformation
Technologies like AI will increasingly become part of everyday life. But sustainable transformation can't happen with technology alone. Instead, it will be up to enterprise leaders to maximize the benefits of data and analytics for organizations, employees, and consumers alike.
As an analytics leader, I'm excited to be part of this evolution.