- Point of view
Intelligent automation: Why you need governance over the new digital workforce
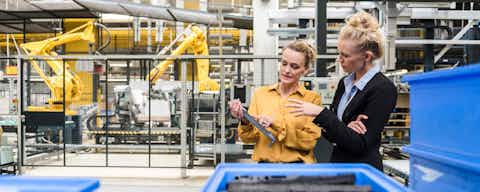
Intelligent automation is the future. You can automate routine tasks and then combine data, analytics, and artificial intelligence (AI) to replicate cognitive thinking. It promises to amplify – not replace – human effort, creating a hybrid workforce wherein bots and people will work together to improve experiences for employees, customers, and partners.
Most intelligent automation initiatives start with robotic process automation (RPA). RPA uses bots to automate routine, rules-based, manual tasks such as data entry or data matching. But as soon as you achieve your productivity goals, it's time to make operations more intelligent.
- Cognitive RPA automates more complex work such as processing application forms or extracting information from a variety of formats. These solutions can work with semi-structured data and derived rules.
- Business process automation digitizes workflows by replacing manual handoffs and paper-based processes to seamlessly connect people, bots, and other systems.
- AI and machine learning can analyze data and identify patterns through supervised and unsupervised learning. This provides people with machine-generated insights, predictions, and recommendations.
As these intelligent solutions gain more influence in the enterprise, trust becomes essential – among internal stakeholders and external customers alike.
To build trust in intelligent automation, governance is essential. It can help you increase machine reliability, monitor performance effectively, mitigate issues, and boost compliance. It also builds trust in new ways of working. After all, intelligent automation is the first introduction many people have to a digital workforce.
Intelligent automation without governance isn't intelligent
When building an intelligent automation strategy, ask yourself – can you check to see if your digital workforce is performing as expected? You need to explain what they're doing, why they're doing it, and prove that they're working effectively with your most valuable resource – your people. Meanwhile, your customers want confidence that their data is in safe hands and that outputs are in their best interests. And, regulatory bodies want to see that this digital workforce is compliant.
Without proper governance, you may open up your business – and your customers – to risks. For example, if banking automation is not configured and monitored properly, it could malfunction and inadvertently expose sensitive client information. Or, it could make a biased decision on a loan application, which could cause reputational damage. It could even become vulnerable to a cyberattack. This would bring huge financial repercussions as well as a loss of the public's trust in the bank, and the bank's trust in intelligent automation.
Take a copy for yourself
To help you get started, here are some questions to ask as you establish a robust governance framework for intelligent automation.
1. Are you automating the right work?
This question is no different from asking if you're giving the right work to the right people. You don't want to assign clerical work to senior managers or management work to clerical staff.
In terms of intelligent automation, you don't want to assign a simple RPA bot to a large, complex process. You want to apply the right type of automation to the right type of work. And, you want to prioritize processes that will deliver the biggest benefits. This requires both process knowledge and automation expertise.
2. Are you using the right type of intelligent automation?
With many automation solutions available, the key is to know which solution caters to which problem. For instance, RPA bots are effective at transactional, user-interface tasks, but encounter issues when a process involves data extraction or paper-based workflows. It's important to construct an intelligent automation solution that can address a broad range of automation opportunities.
At the same time, you need an easy way to analyze tasks to identify an appropriate, corresponding solution. An intelligent automation taxonomy is useful for understanding different types of work – and different types of data – to bring in the right technology at the right time.
Figure 1: Why you need governance over the new digital workforce
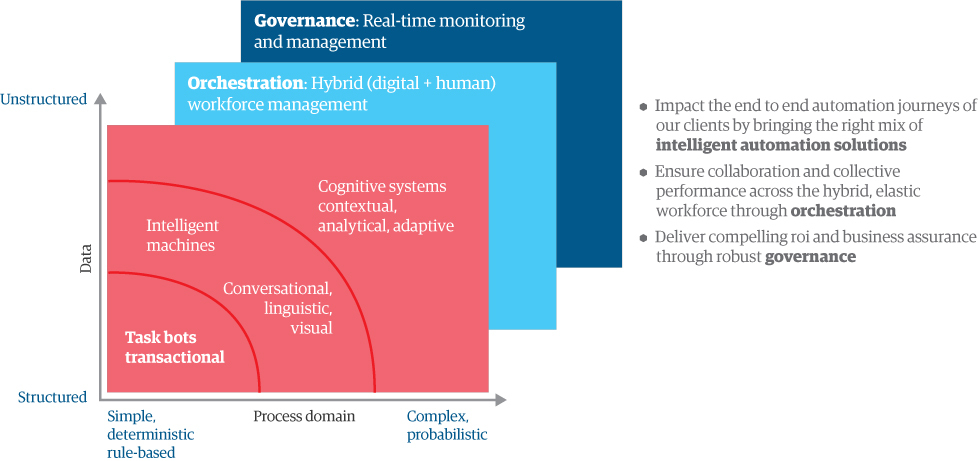
3. Is the work delivered by bots reliable?
You can train, configure, and program bots and other automation solutions according to set rules and a well-defined workflow. But they don't fare well in unpredictable or unknown situations. To prevent process bottlenecks, train bots to handle the complete scope of a task or process across every possible scenario. This requires an automation development life cycle (ADLC) with rigorous controls to build a strategy that delivers reliable results.
4. Is your automation strategy resilient?
Every business is changeable – and its processes are too. A bot can break in unpredictable environments, which is why resilience is critical.
A bot resiliency framework helps bots adapt to changes in user interfaces, data, and workflows to minimize downtime. You can engineer this resilience in a variety of ways, including automated bot monitoring, or even out-of-the-box integration from some RPA platforms.
5. Is intelligent automation explainable?
Not sure why an employee made a certain decision? Just ask. Unfortunately, you can't do the same with bots and AI. Therefore, you need to build an auditable governance framework so that you can explain each step taken to reach a decision. The key is to implement intelligent automation solutions that allow systemic activity logging.
Organizations maintain control and manage compliance by logging 100% of transactions for 100% of processes – securely and in real time. Ultimately, you get an audit trail that can drive compliance, discourage malicious activity, and protect your reputation.
6. Is intelligent automation fair?
With the outputs from intelligent automation directly impacting decisions around your business and customers, you need confidence that they're free of favoritism or discrimination toward certain groups or types of data. An unfair algorithm can lead to unfair outcomes.
For instance, if you're creating an algorithm that assigns spending limits to credit card applicants, your goal might be to treat male and female customers equally. But you also want it to recognize someone with poor credit history. While setting these goals, you can establish risk mitigation protocols to make sure you have well-rounded datasets coming in, and teams ready to spot and correct any instances of unfairness.
Figure 2: Automation breaks
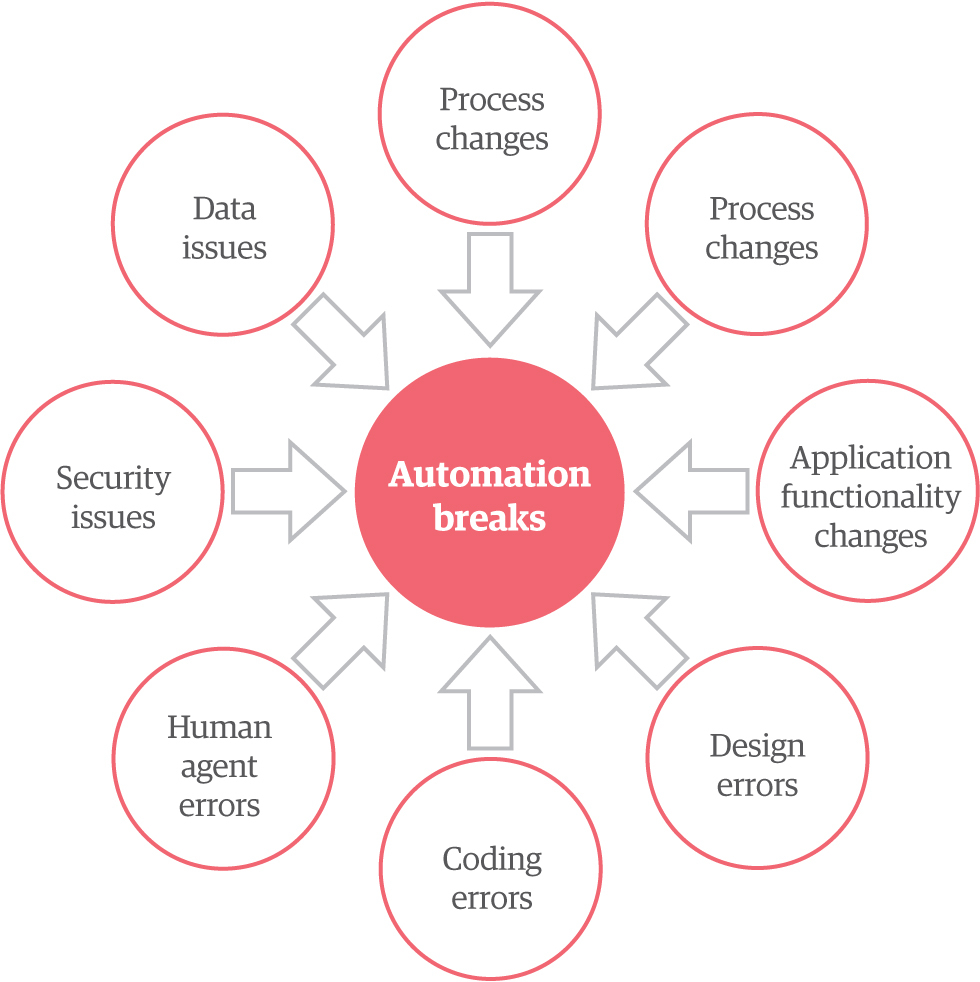
7. How will you measure the impact of intelligent automation?
Enterprises should set key performance indicators (KPIs) for their automation strategy, just as they would for a human workforce. These include direct KPIs, such as process accuracy, speed of handling, and cost savings, as well as indirect KPIs like staff satisfaction, tools replaced, manual labor savings, and error reduction.
8. Is your intelligent automation strategy secure?
Intelligent automation often leverages sensitive data such as passwords, addresses, credit card numbers, and other financial information. Therefore, it's essential to mitigate these risks with a robust security framework that includes:
- Controlled access, protecting privileged accounts across the digital and human workforce
- Protection against sensitive customer and organizational data disclosed by bots and developers
- Traceability, auditability, reliability, and resiliency
- Data privacy by anonymizing data to avoid compromising the privacy and security of a consumer
9. Are your digital workers showing up for work?
In most cases, it's easy to tell when an employee isn't doing their job. In an automated process, you wouldn't know until an output was missing or a process was held up. Therefore, you need a way to spot which bots are at work and which require corrective action. This is where digital workforce management comes in. You can see how digital workers initiate, process, or complete work on a continuous, real-time basis.
10. Is the intelligent automation liable?
A liability framework helps you ensure that the data used to train intelligent automation is only used for the intended purposes of the algorithm. For example, you might create a model from several datasets covering different variables. Then, the AI might take one data stream and use it for another purpose, creating concerns around who's responsible for the outcomes. A liability framework sets parameters around data permissions and intended use.
11. Are bots and humans working well together?
Getting humans and machines to work together successfully is challenging. Define and design this collaboration through hybrid workforce management. Then you can anticipate breakdowns and exceptions, and pass an issue on to the appropriate employee using dynamic workflows.
It's also essential to effectively supervise, monitor, and instruct automated systems. As AI and machine learning generate new insights, you must deliver information to the appropriate decision makers. And of course, people will need training in how to work alongside their new digital counterparts.
12. Is your intelligent automation strategy cost-effective?
One-time costs include technology procurement and development. Recurring costs include infrastructure, licenses, and maintenance. To keep costs down, design automated solutions for stability and low maintenance upfront. Your governance framework should not only track performance, but also the overall cost effectiveness of the digital workforce.
Although the technologies involved in intelligent automation are quite powerful, they are powerless without proper governance. The reward for governance is greater trust, return on investment, compliance, and an intelligent automation strategy that's built to last.