- Blog
Unlock your digital transformation potential with quality data
What's stopping your business from making the most of its data? Perhaps it's data quality.
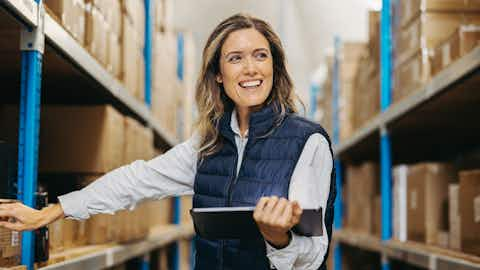
Data is an enterprise's most precious asset. It powers digital transformation, revealing insights for better, faster decisions. But many organizations hesitate to invest in data quality solutions due to the lingering recollection of past expensive projects that failed to prove their worth.
From our experience leading thousands of data projects for Fortune 500 companies, overlooking data quality can result in technology debt – for which short-term inexpensive fixes cause long-term, expensive problems in the future.
And without a solid strategy for data quality, rogue data can impair visibility into operations, derail analytics initiatives, and strain the resources needed to keep processes running smoothly.
In this blog, I'll show you how to create a data quality strategy for gathering accurate and relevant data, improving operations, and potentially saving your company money.
A three-step approach to data quality
To unlock data's full potential, adopting a holistic approach to data quality is essential.
Though some industries have unique nuances, such as regulations around banking and insurance, I've found the following principles apply to all enterprises.
1. Think bigger than data quality tools
Even the best data quality tools are of little use if you don't combine them with industry expertise and solutions tailored to the needs of your business.
Consider demand planners working for consumer goods companies. Inactive products that appear as active in the planning system often lead to inaccurate forecasts, incorrect production orders, excessive procurement of raw materials, and misleading sales projections. As a result, planners need to retroactively adjust their demand forecasts, while business leaders must deal with excess inventory.
On the other hand, by combining data quality tools with industry expertise, you can account for industry nuances for better product management, improving decision-making accuracy, and reducing the need for manual adjustments.
More importantly, by accessing reliable, actionable data, employees can deliver better experiences and meet customer needs more efficiently.
2. Combine data, processes, people, and technology
A comprehensive approach to data quality expands beyond solely focusing on data. It involves examining business processes, developing effective methods to ensure accuracy at every stage, and equipping all employees with the necessary skills for data processing, analysis, and extraction.
Think of data like a river that runs through your organization – to understand it fully; you must look at the sources of the data (upstream) and its effects on people's work downstream. Then, you must evaluate how data collection processes affect the quality of the data and what impact it has.
By considering all four aspects – data, processes, people, and technology – you can identify the most pressing issues to address and understand the overall impact of data quality.
This approach will ensure enterprise leaders get a complete view of the state of their organization's data. And even better, it can ensure employees tap into the treasure trove of data at their fingertips.
3. Recognize data quality as a revenue source, not an expense
To maximize data quality investments, link data quality to your business KPIs. This connection enables leaders to see data quality as a source of value creation rather than a tax.
At Genpact, we've developed a data governance by value framework that helps enterprise leaders define the benefits of quality data. This framework links critical data elements such as time and cost savings to business KPIs, allowing you to measure their potential impact.
Additionally, this approach enables organizations to allocate resources strategically and achieve maximum ROI from their data quality initiatives.
Three steps for cloud modernization
From theory to action: Establishing a Data Control Center
Establishing a Data Control Center (DCC) is the first step toward better data quality.
A DCC combines industry knowledge, an analytics dashboard that presents the size and scale of data quality issues, and a team of data stewards to resolve them. In short, a DCC serves as a control tower, moving data quality functions to the forefront of an organization's operations for chief data officers, chief information officers, and other senior leaders.
Data assessment and visualization tools such as PowerMe trace, track, and evaluate data across the enterprise. Then, with their unique experience and expertise, data stewards turn data into actionable insights, driving positive results for your business.
In summary, a DCC creates a fast track to greater data efficiency, improving the employee experience, supporting better decision-making, and increasing ROI from digital transformation projects.
Powering better ways of working with data quality
To thrive in the digital era, enterprise leaders must prioritize data quality – not just collecting it but establishing and maintaining robust standards. Quality data is essential for creating better ways of working, optimizing operations that lead to cost savings, and enabling emerging technologies like artificial intelligence and machine learning to reach their highest potential.
You can gain an edge over your competition by putting data quality at the heart of your digital transformation journey. And the sooner you start, the easier it will be.