- Blog
Credit and compliance risk: from walls to bridges
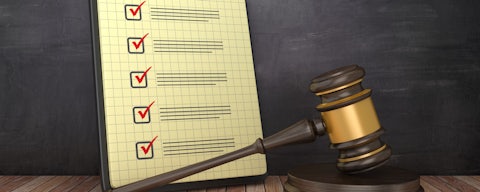
The global financial crisis had a silver lining. It advanced the general state of risk management. And it helped financial organizations realize the benefits of breaking down silos across risk management areas. A prime example? Better coordination between credit and compliance. For financial institutions, faster processing of risk decisions means more business, which drives revenue and growth. Aligning the areas of credit and compliance risk through enhanced data management and advanced digital and analytical technologies enables just that.
Do you know your customer?
Credit and compliance risk managers need accurate, complete, and up-to-date data about their customers. Many compliance regulations, particularly anti-money laundering (AML) regulations, devote themselves to know-your-customer requirements. But for both disciplines, it's important to understand:
- Identity: who the customer is
- Profile: what level of risk the customer represents to the organization
- Structure: the customer's context
- Relationship: the connections that exist between the customer and other parties
There's overlap in the need for this data. So sharing it becomes as important as obtaining it. Fortunately, customer master records containing this data usually exist in centralized systems.
Identity: who is the customer?
You wouldn't trust a stranger. Similarly, credit and compliance risk managers need to gain meaningful familiarity with new customers and parties to transactions, including:
- Name
- Address
- Date of birth (if the entity is an individual)
- Government identification number (such as a tax identification or passport number)
- Articles of incorporation or partnership or trust agreement (in the case of a business)
Financial institutions verify this information primarily through documentation, but other sources can sometimes be useful. For example, contacting a customer by telephone or getting information or references from third-parties, such as credit reporting agencies, public databases, or another bank, can paint a more complete picture. Credit and compliance managers need to have a reasonable belief in the person's true identity, not establish the accuracy of every element of identifying data.
Profile: what is the customer's character?
Identity is important. But financial institutions need to know more about their customers than just who they are. Once identity is established, the next step is understanding the character of that person or company, using reference data that may include:
- Annual income
- Net worth
- Address
- Occupation
- Type of business
- Source of funds and wealth
- Any past misconduct
- Industry
- Geography
- Reputation
This information forms the basis of a risk profile that can be used to assess both creditworthiness and potential suspicious or noncompliant activity. For example, companies that operate in high-risk industries, such as online gambling, may have a higher than normal propensity to default. From a compliance perspective, these same companies may be more likely to engage in illegal behavior. Similarly, both credit and compliance risk managers may flag certain countries as high risk due to social or political issues, the local legal environment, or incidence of corruption.
As the old adage says, reputation counts. Compliance risk managers have always considered reputation to be important. But reputation is also an important factor to consider when assessing a borrower's likelihood to avoid repayment of a debt. An adverse media search, routinely done for AML purposes when high-risk factors are present, can identify reputational risk attributes, such as the presence of a politically exposed person, which can then be shared between compliance and credit.
Structure: what is the customer's context?
Companies don't exist in a vacuum. Another important aspect of truly knowing a business customer is understanding the full legal structure of which it is a part.
In the case of credit, for example, the bank may look to related entities within the corporate hierarchy, such as subsidiary and affiliated companies (including a parent company), for credit support, or need to understand the potential for cash to be transferred from one entity to another. In the case of compliance, the complexity and geographic scope of the legal structure affects the potential for illicit activity to occur within it.
Relationships: how do we connect the dots?
Relationships and connections between parties within the organization are also key to assessing risk. For example, it's important to identify the ultimate beneficial owners, the person, or people with a significant ownership or controlling interest in a company. This is mandated by AML regulations but also needed to assess whether a majority owner might influence a company to act against the interest of creditors, affecting its creditworthiness.
Applying advanced technologies and analytics
Transformation is happening
Technology is transforming the due-diligence and surveillance activities that are key to credit and compliance risk management. For example, artificial intelligence (AI), including natural language processing, can extract data efficiently from the volumes of documents with customer information.
In addition to AI, robotic process automation (RPA), or bots, can conduct pre-transaction due diligence about a customer or counterparty, especially where data-collection activities are repetitive, routine, manual tasks governed by pre-defined rules. Bots can obtain, verify, and process customer data for onboarding and during regular intervals from documents, public databases, government websites, and more. For example, they can retrieve data from regulatory and law-enforcement agencies, such as the SEC, FBI, and Interpol. RPA's benefits include faster and more efficient cycle times, increased accuracy, and freeing up personnel for more productive, revenue-generating, and satisfying work.
Finally, big-data techniques can be used to mine large amounts of data to detect suspicious activity and, increasingly, support predictive models that may flag future credit or compliance issues. In the case of AML, for example, these may be a part of advanced models that generate transaction alerts.
The rise of the middle office
As banks reinvent themselves using technology to drive digital change for the future, risk teams are also doing so. A new tech environment requires new operating models. As advanced technologies and analytics are adopted, institutions are realigning their organizations and rationalize roles to achieve scale, reduce cost, and adopt regulatory best practices.
Tasks that used to be performed manually by credit and compliance teams, including basic due diligence and ongoing surveillance, are being migrated to front-line operational business units, also known as the first line of defense.
As a result, businesses are establishing risk management units that include specialist operating functions – the middle office, to borrow a sales and trading term – that is well-suited to operating these tasks and technologies.
Visit our risk and compliance solutions page
Bridging the divide
Risk managers in different disciplines use different processes to gather data, but the data they need overlap and come from the same sources. Breaking down silos between the credit and compliance risk functions using advanced technologies and analytics yields greater productivity, faster risk decisions, and business growth.
This article was authored by Jeff Ingber, chief advisor to the anti-financial-crime business at Genpact, and Thomas Ruppel, senior advisor to the commercial credit management business at Genpact. The article first appeared in RMA Journal.