- Blog
Augmented intelligence: an untapped opportunity for CFOs
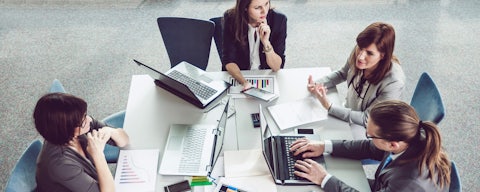
In the age of smartphones, your navigation app is likely to be one of your favorite apps. Whether you're looking for a client's office or the hottest restaurant in town, it will find the quickest route by combining real-time traffic information, road closures, speed limits, and more. And although the app suggests when to turn, you're in the driver's seat. You know when to hit the brakes and make the turns so you get to your destination quickly and safely.
But this combination of machine intelligence and human intuition doesn't only happen on the open road. Artificial intelligence (AI) is helping people work in more efficient ways, leading to augmented intelligence that transforms top enterprises across the globe.
With augmented intelligence, AI processes high volumes of real-time data, connecting the dots, and generating insights. Using these intelligent recommendations — like those of the guidance offered by your navigation app — employees can add years of experience and human expertise into the mix that drives organizations toward the best business outcomes.
Making the most of augmented intelligence
One of the greatest opportunities for augmented intelligence lies in the finance and accounting (F&A) department, which has historically been plagued by mountains of documents, data, hefty number crunching, and tight deadlines. With AI taking over transactional work, finance teams can focus on strategy.
According to our AI 360 study, 53% of senior executives say they're adopting AI for F&A functions. But augmented intelligence presents particular benefits to CFOs.
Helping CFOs make informed decisions
According to the Finance Leaders 2019 Agenda Poll by Gartner, Inc., CFOs rank the following as their biggest challenges:
- Inability to measure business performance
- Outdated financial planning
- Shrinking margins due to inefficient decision-making
- Outdated reporting
- Inflexible financial data structure
If you're a CFO struggling with these issues, what you likely want and need is the ability to clean and collate your data so that you can make more informed decisions. Data-driven insights can steer your company on the path to growth, and augmented intelligence can guide the way, highlighting new opportunities. Equipped with these insights, you can become more of a strategic partner to your organization.
Making augmented intelligence a reality
So, how can you take augmented intelligence from a promising concept to a working reality? Start with the essentials:
- Begin with robust data management: Data is the foundation of augmented intelligence. You need centralized data so that different functions can pull from the same, consistent datasets. You also need people with data engineering and master data management skills who can make sure your data is accurate.
- Create a multi-skilled workforce: For augmented intelligence to work, “bilingual" talent – people who understand both the financial and technical sides of the coin – will be vital, as they can look at AI-powered models from a business context. If you identify missing skills, make sure you prioritize this when both training existing employees, and hiring new employees.
- Take control and govern AI: Our AI 360 study shows that 78% of consumers expect companies to actively address potential biases that can negatively impact AI outputs. Biased data can skew decision-making and elevate risk. You need to be sure that you have current, holistic datasets when training your models, and governance to watch out for unwanted influence. Governance also means having traceability for how a machine came to its decision or recommendation. Traceability prevents AI from becoming a “black box" in the eyes of consumers and regulatory bodies.
- Plan for change management: Working with machine intelligence presents a big change for longstanding F&A teams. You can minimize resistance among teams by communicating how AI enhances their day-to-day functions in taking over transactional work – e.g., the statistical calculations involved in financial forecasting. Moreover, provide reskilling opportunities so that teams can combine their expertise with AI for more value-added activities, such as identifying new revenue streams. CFOs can also set an example by using machine intelligence to guide strategic choices that align financial functions with business goals.
- Consider pretrained accelerators: Deploying AI can take time, but you can speed up adoption using pretrained AI accelerators. At Genpact, these accelerators are built with the expertise we've gained from years spent working with global businesses, so that they draw on insights that are unique to your industry and function. Rather than redesigning entire systems, you can plug them into your core business processes using a modular approach, and start taking advantage of augmented intelligence sooner.
To learn more about what's possible with augmented intelligence, read our latest AI in F&A point of view.