- Point of view
Reimagining advertising with digital: Connecting buyers and sellers
Technologies including robotic process automation, AI and natural language processing (NLP) can bring accuracy and speed into ad operations
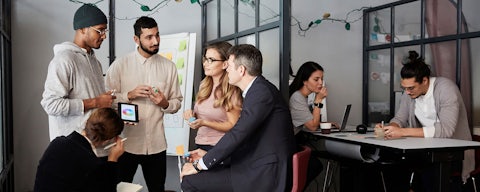
After successfully applying automation and artificial intelligence (AI) to finance and IT, media companies are now considering how digital interventions can better serve core media functions such as ad sales and ad buy. Traditionally, these highly manual processes have depended on human judgment — and when that judgment falters, revenue suffers. Additionally, with the current shift from linear to digital ads, middle and back offices must keep up and make their processes smarter with digital interventions, including robotic automation, AI and automated workflows.
Most media companies have taken significant steps with digital, using automation and artificial intelligence (AI) to make their finance and IT functions more efficient. But there's more work to do. In today's rapidly evolving media landscape, successful advertisers must identify and pursue highly specialized audiences on every available channel. As well, they demand clear evidence of a campaign's success. Yet some firms still depend on yesterday's inefficient ad buy and sell protocols, making them less competitive — and less profitable.
That's unacceptable, because whether you're a publisher selling ads or an agency planning and placing them, boosting revenue is the goal. But the longstanding manual processes most media professionals follow make that objective difficult to achieve. The reason: many legacy operations rely on dated applications and Excel spreadsheets that leave far too much room for mistakes, aren't scalable and take up excessive time. Too many touchpoints and hand-offs in the system also create vulnerabilities. What's more, all too often the middle and back office don't play well with the front office, which typically is more digitally advanced.
Maneuvering in a complex environment
From publishers, there's an ever-growing number of offerings, including linear, direct response, on air or digital advertising products. Choosing the right mix calls for deep intelligence. Sellers must monitor newly-evolving consumer trends and customer needs — a powerful way to produce the tailored proposals that will win over new clientele and maintain their base. And they want to be confident that their pricing is competitive. But cumbersome procedures for buying and placing ads aren't agile enough to respond to a changing marketplace in real time.
The traditional way of doing business is hampering agencies too. For instance, when on-air ads get bumped for special programming or audiences are smaller than expected, the campaigns they've designed may not meet the set goals or KPIs. As a result, the piecemeal manual strategies they put to work can burn up an ad budget in no time — leading to dissatisfied customers.
Insights from a wealth of data
Today's digital technologies address issues for both parties. Automation can now administer, service and fulfill ad orders — but it can do much more. Robotic process automation, AI, natural language processing (NLP) and smart workflows can bring accuracy and speed into ad operations, generating revenue growth. Digitization also produces a wealth of data and insights from analytics — intelligence vital for superior campaigns — and helps sellers and buyers with intelligently managed dynamic pricing strategies.
The efficiencies automated processes deliver cut costs — a plus in itself. But new approaches also improve the user experience for internal and external stakeholders alike. And when machines take over mundane, error-prone tasks, such as entering orders and following them through to completion, staff are free to add greater value to their enterprises. For example, some firms are upskilling their linear ad teams by teaching them the ins and outs of digital ads. That's where the market is growing — so the move not only advances their careers, it also enhances the bottom line.
Integration for publishers and agencies
The key here is that digitization integrates the ad process from publisher to agency every step of the way. Both sides benefit when everything communicates and works in sync — opening the door to even more targeted digital interventions that bring additional benefits.
For example, using NLP and AI, they can extract data from purchase orders and feed it directly into the order-management system, creating real-time integration with the inventory system. Smart workflow orchestration can manage and direct the deluge of emails between sales, operations and traffic teams, and analysis of ad-lifecycle data can build models for dynamic pricing.
Genpact has helped radio networks, studios, publishers and agencies transform the way they sell and place ads by combining process optimization and digital technologies. For these media companies, the impact of transformation on productivity tells the tale: We've seen it go up by 40 percent to 50 percent — and that significant measure is just a taste of the benefits to come.
This article was first published in M&E Journal.