- Blog
Straight through claims processing: The launchpad for insurance automation
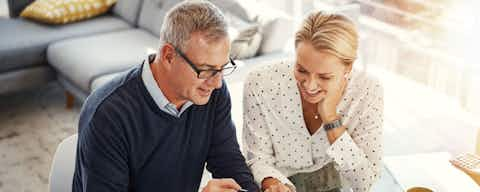
Over the course of the COVID-19 pandemic, we've all experienced major changes to the way we live, work, interact, and expect services to be delivered. Insurance carriers have risen to the challenge of their workforces having to service customer needs remotely. From a claims servicing perspective, this has meant a reduced ability to inspect damages in person and pressure from customers to provide more streamlined processes and claims automation as they juggle work and family commitments at home.
These changes and demands have prompted insurers to race toward viable digital and automated interventions that make the claims process quicker and easier for their customers with fewer manual touches and improved efficiencies. But they've realized that low adoption of automation is hindering these goals. According to LexisNexis and Salesforce, claims automation could potentially free up to 54–285 million claims adjuster hours every year, amounting to cost savings of $1.7–$8.9 billion within five to seven years. But these huge cost savings aren't the only positive – the automating claims process brings benefits across the entire claims organization (figure 1).
Figure 1: Impact of claims automation
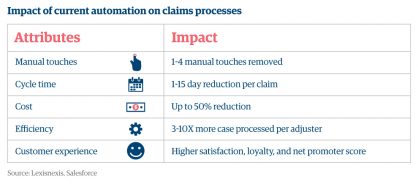
The benefits of straight through processing in insurance
One of the key parts of an insurer's automation toolkit is to build out and embed straight through processing (STP) across the claims lifecycle. By implementing true STP automation in key claim activities (eg, FNOL, document management, customer communications, fraud and subrogation predictive analytics, and payments) and across certain claim types, carriers can give customers a highly prized self-service ability, enhancing the customer experience and removing unnecessary manual touchpoints for a more efficient claims process.
STP can even make certain claims a no-touch or low-touch end-to-end process. Fast track claims (minor damage claims) are ripe for this type of intervention. By using STP for these claims, adjusters can then focus their skills on higher-dollar impact claims to ensure proper investigation and resolution. When a carrier begins to use STP interventions in fast track claims, they can start migrating some of the key elements of this claims automation (for example, automatic segmentation and triage, auto reserving, and customer notifications) across other claim types to boost efficiency, increase standardization, enhance the customer experience, and reduce costs. Figure 2 shows an example of how STP in fast track claims can work.
Figure 2: How STP works in fast track claims
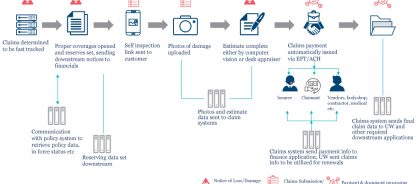
Getting started with straight through processing
For a carrier to successfully implement STP, it's crucial to identify gaps and issues in its current process flow. We carry out diagnostic and blueprinting exercises with our insurance clients to uncover which areas are suitable for claims automation and digital interventions to drive STP.
One of the areas in which a carrier can begin an STP redesign is in low-complexity, fast track-type claims. Regardless of carrier size, they can all automate these types of claims. These claims typically have fewer coverage issues and do not require manual adjudication interventions to drive them to settlement. Carriers can quickly reap the benefits of automation by moving these low-complexity claims to an STP process to shorten cycle time, improve accuracy, and provide additional capacity for adjusters and other internal staff.
The pandemic has changed how customers expect their claims to be serviced. Now, customers will expect these more automated, self-serve claims processes to continue, and STP is an obvious choice for streamlining processes and keeping customers happy.