- Report
Realizing the benefits of artificial intelligence with machine learning operations
Discover a new approach to implementing AI and machine learning technologies
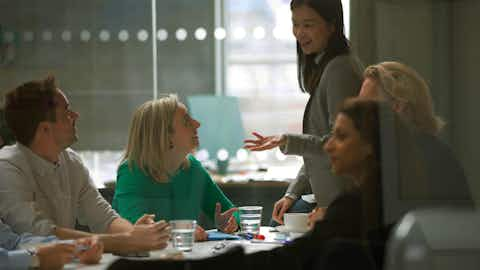
Across industries, enterprise leaders are ramping up digital initiatives at an unprecedented pace, reinventing how we live and work. But as these projects get underway, we've witnessed that roughly only half of all AI proofs of concept ever make it to production. Indeed, for most teams, achieving their AI vision remains a distant reality.
To help organizations maximize the value of their AI and machine learning (ML) investments, we've developed a playbook, uncovering insights from our experience running thousands of processes. In partnership with NASSCOM, a think tank in tech, we've outlined a robust methodology that helps unify people, processes, and technologies across the enterprise.
At its core, machine learning operations (MLOps) helps teams consistently develop, deploy, monitor, and scale AI and ML models, mitigating the potential risks associated with not having a framework for sustainable innovation. Think of MLOps as a formula for success.
Every journey needs a guide. And when it comes to adopting AI and ML at scale, we believe MLOps can be your North Star.
Discover how to deploy AI at scale
Explore more digital transformation insights
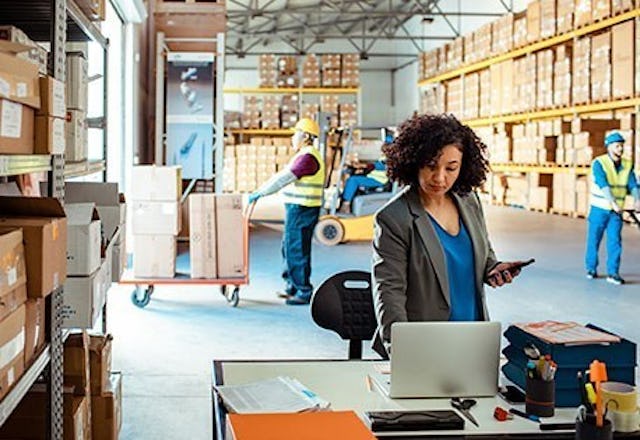
MLOps best practices for your industry
Discover how enterprise leaders across industries use MLOps to drive innovation at scale.
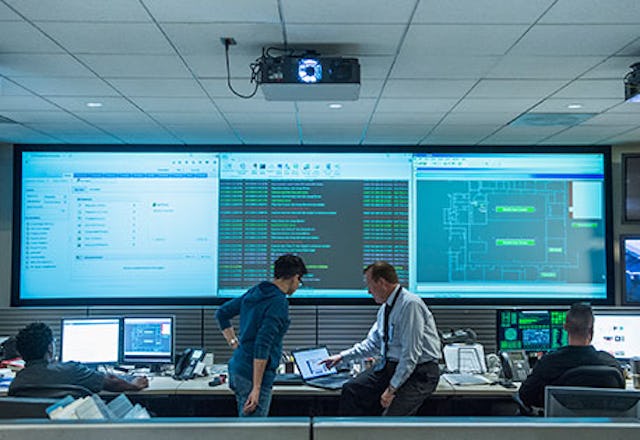
Understanding your MLOps maturity
This handy guide helps you assess where you stand in your MLOps journey.
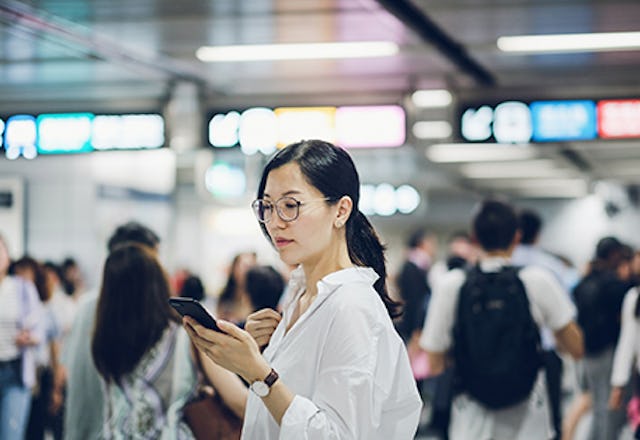
Responsible AI: Developing technology for everyone
Learn how to build safe and reliable products with AI.
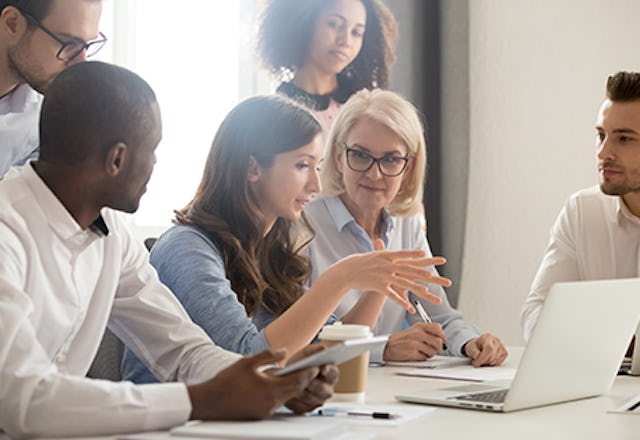
The upskilled workforce
Learn how to train teams to generate data-driven insights at scale.
Meet the instinctive enterprise
The next generation of successful businesses will connect, predict, and adapt at speed with AI as their neural wiring.
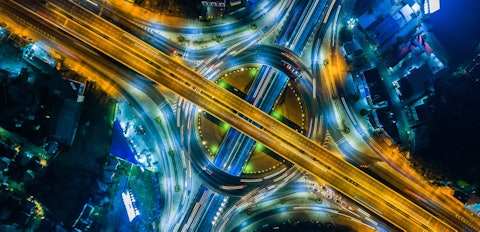