- Point of view
The right AI can hone revenue forecasting for better business decisions...
...and make finance a real partner in the enterprise
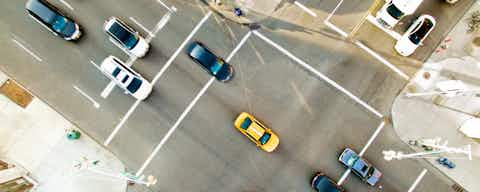
AI dominates most of the talk in today’s enterprises when it comes to technology. We’re always finding new ways of using AI to generate better business outcomes. But the jury is still out on how AI will affect our work and life in the future.
In the financial context, though, one question often comes up in conversations with our clients: How to arrive at the right short- and long-range revenue forecasts. Revenue projections have far-reaching implications because they drive annual budgeting and planning cycles. When forecasts are inaccurate, firms budget and invest inefficiently, making it harder to meet targets and leading to poor financial performance.
That’s why, for effective decision making, any AI forecast methodology must be intelligent, agile, and able to accurately reflect market dynamics. For agility, it should decode relevant signals from the structured and unstructured data it gathers from internal and external sources — and factor them into projections. To improve accuracy, it should analyze what causes the variance between forecast and actual numbers, continually learning on the job.
Until a few years ago, all this could only happen in a lab under controlled conditions. But AI has made such giant strides that we can now deploy sophisticated systems at scale.
Let’s examine exactly how AI aids strategic decision-making in finance through better revenue forecasts.
The key forecasting challenges
Most companies manually perform forecasting using Excel spreadsheets that require input and involvement from sales, finance, and operations, among other departments.
Not only can this disrupt business as usual, the forecasting methodology isn’t uniform across regions. What’s more, the assumptions people make are frequently subjective and biased. This means forecast accuracy can vary between 50% and 80%. Moreover, with all the data and methodology variability across markets, management doesn’t get a consolidated global view. Finally, because of the major effort involved in the overall exercise, companies only refresh numbers annually. That doesn’t align closely with the decision-making cycle.
Bringing method to the madness
Today, however, machine learning algorithms can completely automate the forecasting process, cutting down on cycle time and effort. These algorithms can run on demand to generate accurate business performance forecasts and provide early warning signals. With this forward-looking intelligence in hand, an agile management team can offer guidance to the street with increased confidence and can allocate scarce resources appropriately.
A case in point
Consider the following example. A global telecom major was struggling to improve the accuracy of its revenue forecast, which relied on biased sales estimates. The process was manual and the one-size-fits-all method didn’t consider market nuances associated with each product line. This was giving rise to more than a 10% variance between actual and forecast numbers.
Then, Genpact used its deep domain expertise to identify the lead indicators and key input variables. We applied machine learning techniques to create a robust forecasting model for each product. We automated monthly forecast runs by coupling machine learning models with a data engineering solution to manage input. We also set up an advanced visualization platform to deliver timely insights to the business.
As a result, forecast accuracy improved by 50%, variance decreased to less than 5%, and the company reduced its forecast generating cycle by about 90%.
What are the key components of this AI solution?
- Domain: First, it’s critical to identify and map the factors that have a material bearing on revenue. Some common drivers are sales projections, competitor activity, pricing variability, marketing and promotions, and incentive programs. You may also need to carefully consider other issues, such as macroeconomic indicators, industry-specific nuances, and regulatory obligations.
- Data Science: Then, machine learning models link those inputs to the output — revenue. Supervised and unsupervised learning techniques allow for continuous improvement in the forecast accuracy.
- Digital: Data engineering and management next makes sure the right data is available for the forecasting model. Digital technologies such as text mining and natural language processing (NLP) process unstructured data. Advanced visualization techniques drill-down by region, market, and brand. And natural language generation (NLG) automates commentary accompanying the forecast numbers.
Selecting the right partner to get the most out of AI forecasting
Here are a few things to consider.
The ideal partner needs the relevant domain expertise and depth to identify and map the crucial drivers that influence revenue, at an industry and company level.
In addition, the partner should have strong capabilities and experience in delivering a full spectrum of advanced analytics services, including predictive analytics, big data, data engineering, business intelligence, and reporting.
Finally, the partner needs to have the proven ability to drive digital transformation in business operations using intelligent automation, artificial intelligence, and analytics.
This point of view was authored by Ruchita Niyogi, Assistant Vice President, Enterprise Performance Management at Genpact.