- Point of view
Mastering your pricing analytics strategy
Combining machine intelligence and human judgment to drive growth
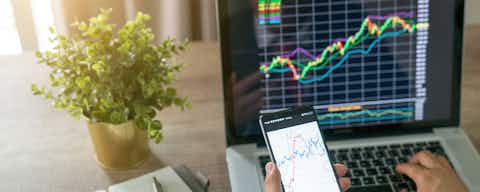
A clear, effective pricing strategy underpins profitable business growth. Unfortunately, developing such a strategy can be complicated. Price too high and pressure grows to secure a high volume of sales to protect profits. Price too low and risk damaging perception of the product, service, or brand – along with margins.
To find the optimum yet competitive price, your pricing strategy needs to balance buyer expectations, budgets, perceived value, and internal and external influences. With so many factors at play, purpose-built analytics can offer decision makers a lifeline.
Advanced analytics can generate pricing recommendations based on competitor insights, evolving market trends, real-time sales velocities, customer behaviors, and changing market conditions.
When decision makers take automated insights into their hands, they can apply their knowledge and experience to take swift, decisive action. The result – augmented intelligence – is a blend of machine intelligence and human judgment that guides decision makers toward the ideal price at any point in time.
Harnessing the power of pricing analytics
Pricing analytics can reduce risk, boost sales, and increase margins – even in the face of market disruption. In fact, with effective pricing, enterprises can often improve profit margins by at least 0.5–1%. So, what does this look like in practice?
One aviation leader identified a $30 million margin gap against target. The company had previously set prices for its spare parts without considering the connections between prices, buyers, and perceived value. Working with Genpact, the company found that its customers would make buying decisions based on how they use, consume, and replace different parts. This formed the basis for the company's decision to map part pricing against perceived customer value. With this approach, the company formalized a pricing strategy, created trackable pricing lists, and developed a dynamic pricing model that resulted in a $60 million margin improvement.
Similarly, an oil field services provider didn't have a standard list of prices for its products, rentals, and services. This led to disparity across customer quotes and negative perceptions about the company's pricing. Genpact helped the company build machine learning-based algorithms to generate standardized prices according to region and product. Supported by advanced visualization dashboards, the company improved profit margins by 2–3%.
Mastering your pricing analytics strategy
As you begin to look at your own pricing strategy and analytics capabilities, there are many different areas to consider. Based on our experiences, we believe these are the highest priority:
- Define success: There's no single price that will deliver optimal results across all KPIs. So, you need to determine and prioritize the KPIs that are the greatest indicators of success or most relevant to your business objectives.
- Price by customer segments: In a perfect world, pricing would be optimized for each individual customer. But that can lead to errors and discrepancies, especially if customers share quotes. To find a balance, segment customers with pricing personalized to each group.
- Segment dynamically: Remember that customers tend to shift between segments over time. If you consider the customer journey in your pricing strategy, you're more likely to secure customer loyalty as they move from early adopters to frequent buyers.
- Implement lifecycle pricing: Product lifecycles vary throughout your customer base. Some customers perceive that a product is in an introductory phase while others feel it's mature and worth a premium. Therefore, you should price for different customer groups at specific points in time.
- Review your models: Typically, companies model price against product complexity and a range of features. But features change, some products don't achieve their expected sales volumes, and external influences can give products a limited shelf life. Establish a governance process to regularly review your models against the products you – and your competition – offer and make changes to ensure prices are always competitive.
- Customize your data and variables: Many companies rely solely on the most convenient datasets when conducting pricing analysis. But for effective pricing analytics, you need the best available data. Make sure you have a strategy for tailoring your pricing evaluation process to harness the most appropriate datasets and variables.
- Build for reusability: Because pricing is not a one-off task, you should build your methods and models for reuse. Machine learning frameworks can automatically refresh model development and pricing recommendations to maximize reusability.
- Don't forget psychology: Beyond the analytics of determining a price, understanding the psychology of pricing and the customer is key. For instance, continuous exposure to higher prices can reduce customer sensitivity to the price of a product.
Across all these areas, it's important to consider the input of team members with relevant industry expertise and experience. If their intuition tells them a price is too high or low, they should explore this sentiment and use it to continuously improve the models and pricing recommendations.
Augmenting intelligence in optimized pricing and analytics
Pricing decisions could be made entirely by algorithms or entirely by people. But the optimal pricing decisions lie between these two extremes – where pricing analytics plays a crucial role.
For example, when a salesperson decides to deviate from a recommended price to close a deal, they call upon all the customer insights available. When contract prices are set, they're based on expected long-term revenue and related insights. When commodity product prices are determined, they're decided based on margin, profit, and turnover expectations. All these decisions are not merely human decisions, but decisions that analytics can and should augment.
Finding the optimal price is not just a mathematics problem, it's a decision-making problem. And it's a decision that is sometimes bound in politics, emotions, and economics. Therefore, human intelligence combined with purpose-built analytics often leads to the best outcomes across the board.
With an effective pricing analytics strategy, the optimum price for every product and every customer can be identified. This means – even during periods of disruption and uncertainty – that confidence in pricing decisions can protect profits. It's the best of both worlds: human and data-driven insights.