- Blog
Preparing for 2025: Three trends shaping the future of high-tech manufacturing
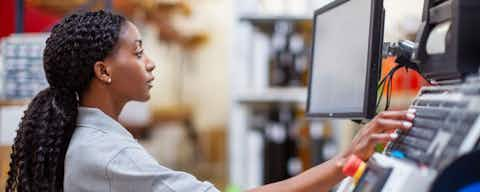
Digitization, servitization, customization. These priorities come up time and again when talking about the future of high-tech manufacturing. Are you ready to adapt?
The need for digitally led business models in manufacturing is clear. COVID-19 increased consumers' thirst for technology that unites people across borders and created a surge in demand for health-boosting tech and medical devices.
It also revealed a lack of resilience and adaptability across supply chains. Some of the biggest names in the industry have warned of loss of earnings because of a downturn in demand for core products combined with increased operational costs. Across the board, 67% of global electronics manufacturers and suppliers report that they're extremely concerned about the impact COVID-19 will have on their business.[1]
Becoming an instinctive enterprise
While facing these challenges, there's an opportunity for transformation. To help you get started, Genpact's Manufacturing in the Age of Instinct report introduces three macrotrends shaping the future of the world and the manufacturing industry.
In this blog, I look at what these macrotrends mean for high-tech manufacturers and how they can become instinctive enterprises. The instinctive enterprise is the next generation of successful businesses that seamlessly connect people, processes, knowledge, and judgment to make accurate, proactive decisions in real time. They will move markets, reinvent business models, and amplify human potential.
An instinctive enterprise will navigate these three macrotrends:
1. Optimized reality
The optimized reality trend is about blending technology and people to create augmented workforces that can deliver hyper-personalized services and experiences.
High-tech manufacturers can make this vision a reality by embracing the internet of things (IoT) connectivity. Hybrid human-machine production lines will also be essential, especially for operations in which accuracy and customization are key.
Manufacturers must also develop capabilities for more adaptive supply chains to deliver personalized services at speed. According to recent predictions, there will be 94 million machine vision systems – technology used to provide imaging-based automatic inspection and analysis – in industrial manufacturing by 2025.[2] This technology, combined with AI, will enable manufacturers to continually monitor and optimize production.
High-tech manufacturers should develop production lines made up of flexible cells that can reconfigure themselves as necessary to quickly adapt to peaks and valleys in demand. Automation and 3D printing will help make production more customizable, localized, and demand led.
2. Ethical impact
The ethical impact trend pushes manufacturers to control standards and sources to meet customer expectations for companies to be ethically and environmentally responsible.
Even during periods of disruption, environmental impact cannot be compromised. Semiconductor fabrication plants use a huge amount of energy, while digital technologies have the potential to reduce current global carbon emissions by up to 15%.[3] In every business, there's a need to evaluate energy and resource optimization throughout operations.
Ethical practices also extend to how manufacturers care for people. Apple's global supply chain introduced health screenings, social distancing measures, deep cleaning protocols, staggered shifts, and mandatory personal protective equipment (PPE) at speed and scale to protect workers from COVID-19. Now is the time to explore how automation technologies, such as industrial IoT and collaborative robots, can make these practices easier to manage and more sustainable in the long term.
3. Whole-system planning
Finally, the whole-system planning trend encourages manufacturers to own less and explore new partnerships to unlock and realign the capital required for service-led business models.
In the wake of social, environmental, and political uncertainty, businesses should work together – even with competitors – to boost resilience and protect profits, especially as new competitors such as Facebook enter the semiconductor market.
There's also an opportunity for manufacturers to streamline production with innovative tech. For example, high-tech manufacturers could partner with companies using extreme ultraviolet lithography – which uses very short wavelength light to construct fine patterns – to make powerful microchips in less time and at less expense.
As the manufacturing industry prepares for the future, every high-tech manufacturer can become an instinctive enterprise. Though disruption is unwelcome, it can also be the catalyst for developing new and better ways of doing business.
To explore the trends shaping the future of manufacturing in more detail, read the Manufacturing in the Age of Instinct report.
Visit our Manufacturing in the Age of Instinct page
1. https://www.statista.com/statistics/1106047/electronics-manufacturers-level-of-concern-about-covid-19-impact-world
2. https://www.prnewswire.com/news-releases/installed-base-of-machine-vision-systems-in-industrial-manufacturing-to-reach-near-100-million-by-2025-301002034.html
3. https://www.weforum.org/agenda/2019/01/why-digitalization-is-the-key-to-exponential-climate-action/