- Podcast
Journeys in Transformation - Gaining a competitive advantage with augmented intelligence
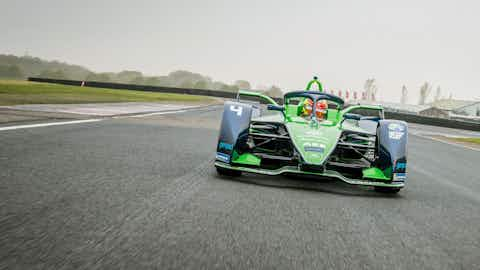
At its core, augmented intelligence enhances human decision-making with AI-powered analytics. And for Envision Racing, a founding team in the world's first fully electric, international single-seater street racing series – the FIA Formula E World Championship – augmented intelligence has redefined how the team wins on and off the racetrack.
Join Genpact's global analytics leader, Amaresh Tripathy, and Sylvain Filippi, managing director and chief technology officer of Envision Racing, to learn how our analytics solutions help the team enhance fan engagement, reduce carbon emissions, and defeat the competition.