- Point of view
Data: The lifeblood of digital transformation
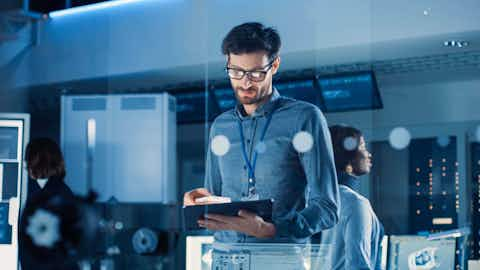
All employees make decisions that impact how you do business and serve customers. But how do you know that they're making the right decisions? Decisions that will help your business innovate, create value, and thrive. You need a culture of data-driven transformation, in which data-driven insights empower employees to make the right choices at the right time.
The journey to data-driven transformation
Successful enterprises tap into their treasure troves of data to stay ahead of the competition. They use data-driven insights to take decisive action and save time and money on digital transformation projects that could have taken months or even years.
Unfortunately, many enterprises are unable to kick-start data-driven transformation. But for those that do, the results speak for themselves.
Data-driven transformation helps business leaders understand customer behavior and identify opportunities to develop innovative solutions and services for employees and customers. It uncovers solutions for some of their biggest challenges.
Data-driven forecasting bears fruit
A global seller of fresh berries with a network of more than 750 growers worldwide was struggling to process harvest orders, manage sales pipelines, and assess market demand. By creating a data-sharing app for growers and retailers, Enquero – a Genpact company – helped the seller unlock real-time insights into sales and demand. This solution gave the seller the ability to scale operations when needed and act faster as market demand fluctuated.
For enterprises that want to embark on a data-driven transformation journey, there are four steps to follow:
- Embrace augmented intelligence
- Diagnose and improve outdated processes
- Harness the power of the cloud
- Embed data governance, security, and compliance
1. Embrace augmented intelligence
Augmented intelligence is the future of decision-making, and data-driven transformation can't happen without it. But augmented intelligence needs to apply within industry context – the approach adopted by a bank will differ from the approach adopted by a retailer.
This industry-led approach is possible through partnerships with analytics experts who understand an industry inside and out. It helps enterprises avoid applying technology for technology's sake and make the most of the data-driven insights uncovered.
Case study
A vision driven by data
A Formula E team used augmented intelligence, combining advanced analytics and human input, to stay ahead in the FIA Formula E World Championship. With advanced analytics, engineers and drivers gained previously unreachable insights from the drivers' practice runs in the simulator and on the track so the team could perform at its best. In motorsports, augmented intelligence helps the team make confident data-driven decisions about energy choices, overtakes, and defensive moves to secure a podium finish.
2. Diagnose and improve outdated processes
Data-driven transformation isn't about applying innovative technologies to ineffective processes. Its value is in identifying existing inefficiencies for correction before building more effective and sustainable solutions.
Most enterprises don't know why processes aren't working as they should. And it's understandable – getting to the root of the problem is a time-consuming task without data-driven insights.
Consider the data-science technique of process mining. When applied correctly, it creates a digital copy of a process to pinpoint inefficiencies. For business leaders, this type of insight is what drives lasting data-driven transformation.
Case study
Predicting and adapting at Panasonic
For electronics giant Panasonic, a lack of data visibility across its complex supply chain led to low demand forecasting accuracy, blocked working capital, and rising inventories – until it partnered with Genpact. We worked with employees to understand the challenges they faced and the root cause of them. Then, we developed a solution to make augmented intelligence a reality. A new data-driven approach enabled employees to make supply chain forecasts daily instead of monthly – all while improving accuracy.
3. Harness the power of the cloud
There's another piece of the data-driven transformation puzzle to consider: the cloud.
Cloud technology allows enterprises to gather and analyze data from multiple internal and external sources. Then, business leaders can efficiently collate, cleanse, and explore vast amounts of reliable data to make more informed decisions.
Just think of how the homepages of Netflix, Amazon, and Spotify use data-driven insights to adjust recommendations daily based on users' preferences. Many customers don't even realize cloud technology is part of the process. All they care about is the experience.
This data-driven approach to transformation can create memorable experiences for customers. But it begins with employees. Employees must be equipped to harness the power of data and analytics in the cloud. With quick access to meaningful insights, they can deliver the exceptional service customers expect.
Case study
Taking decisive action in healthcare
A healthcare solutions company needed streamlined oversight of its finance and supply chain data to reduce reporting time, standardize performance metrics, and improve decision-making. We used a cloud-based data engagement platform to harmonize data across systems. The outcome is a single dashboard that gives finance and supply chain employees access to predictive and prescriptive insights so they can make real-time decisions with confidence to deliver a better customer experience.
4. Embed data governance, security, and compliance
An often-overlooked transformation challenge is the efficient, secure, and ethical management of data – especially for enterprises in highly regulated industries like financial services.
Prioritizing security, compliance, and removing potential data biases is crucial. This effort requires a concerted alignment across the enterprise as part of an overarching data-driven transformation strategy. It means creating clear guidelines for data management and upskilling employees for increased data literacy with ethical usage and regulatory compliance top of mind.
When data, people, processes, and technology align
Ultimately, an enterprise's ability to embed data-driven transformation at every level of an organization will determine its survival in an increasingly data-driven world. Change happens fast, and employees need data-driven insights to adapt accordingly and make the right choices every time.