- Point of view
The future of decision-making: Augmented intelligence in business
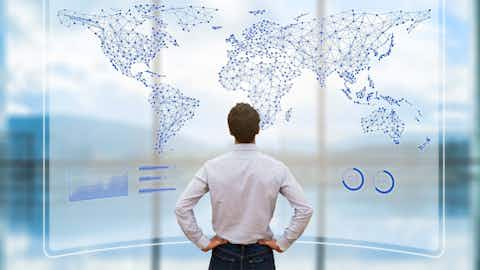
What happens when you combine data, analytics, and artificial intelligence (AI) with human knowledge? Augmented intelligence – where AI's analytical power and speed take over most data processing tasks, producing data-driven insights that help guide decision-making. Combined with advanced analytics, automation, and cloud technologies, augmented intelligence has transformed how enterprise leaders and their teams keep up with the rapid pace of business disruption. So, how can you realize the benefits of augmented intelligence? Here we outline some of the steps you can take.
What is augmented intelligence?
At its core, augmented intelligence enhances human abilities through the power of machine-generated insights.
In modern aviation, for example, a pilot relies on systems computing mountains of data to assess navigation routes, weather patterns, and other critical details. With this information and their flying experience, pilots can make sound decisions. Similarly, business leaders rely on augmented intelligence solutions to streamline business functions, uncover trends, and unlock ideas to increase compliance, improve the customer experience, and boost revenue growth.
As technology continues to evolve, so will the use of machine intelligence across industries. With its capacity to capture human knowledge and continuously build upon those hard-earned learnings, augmented intelligence has the potential to revolutionize how we live and work.
But technology alone can only go so far. In other words, business leaders must connect augmented intelligence to the unique needs and nuances of their enterprise. With a tailored approach that combines people, processes, data, and technology, organizations can reap the full benefits of augmented intelligence, opening the door for endless transformation opportunities.
Creating a recipe for success
Think about augmented intelligence like fine dining. Data and digital technologies – such as AI and machine learning (ML) – are the ingredients, but experienced chefs need to combine them in the right ways and at the right time to make an outstanding dish. The chefs in this scenario are your data scientists, data engineers, and professionals with industry expertise.
Similarly, technologies such as cloud-based architectures can help your teams connect all data sources in place. Of course, you can plug data into AI to create new models and uncover predictive recommendations, but these models do not exist in a vacuum. They have inputs and outputs that impact the rest of your business, which you must closely govern – so it's critical to build a reliable foundation that can support long-term innovation.
Likewise, you must have a holistic view of how you plan to maintain and improve your strategy – helping humans and machines to work better together.
Predicting and adapting at Panasonic
Electronics multinational Panasonic struggled with a lack of data visibility across its complex supply chain, negatively impacting demand forecasting accuracy and employee productivity. That was until we deployed a full stack of advanced analytics capabilities built on Genpact Cora, our augmented intelligence business platform.
First, we worked with employees to understand their challenges, wants, and needs. Then, we developed a decision-support engine and data visualization tool on the cloud that gave employees visibility of end-to-end supply chain data. This achievement meant they could forecast daily instead of monthly, improving forecast accuracy by up to 75% while freeing up 30% of the working capital tied up in inventory.
The secret ingredient of augmented intelligence: People
With the rise of AI, automation, advanced analytics, and the cloud, the augmented intelligence industry has experienced a short supply of a key ingredient – people. Specifically, data science professionals.
You're likely to find two skill sets among this group: industry experts with deep business knowledge and technology experts well versed in data, analytics, or AI. The challenge is finding professionals with both industry and technology expertise. This combination is crucial when implementing augmented intelligence into core business processes.
Some data scientists, for example, can spin extensive webs of data into theoretical models but often struggle to transfer those projects into production. Or worse, they cannot scale those solutions across an enterprise. Similarly, developing a successful analytics model would be nearly impossible if a project has 15 industry experts but no data engineers. And that's why this combination of technical and industry expertise is vital.
A new approach to advancing sustainability goals
After a long period of disruption and uncertainty, customers, employees, partners, and investors increasingly expect organizations to focus on long-term value creation. With this new mandate, leaders have turned to augmented intelligence to build resilient, purpose-led organizations that prioritize communities, employees, and the environment alongside customers.
With augmented intelligence software, enterprises can identify opportunities to:
- Deliver exceptional experiences and services
- Improve equality, diversity, and inclusion in the workplace
- Reimagine supply chains to minimize disruption and waste
- Reduce energy usage, cut carbon emissions, and meet climate-action commitments
And seizing these opportunities is paying off. Research shows that environmental, social, and governance (ESG) initiatives can drive significant competitive advantage, innovation, and financial performance. By prioritizing these efforts, enterprises can also stay ahead of new and changing regulatory standards.
First steps toward embracing augmented intelligence
As you develop your augmented intelligence strategy, consider these steps:
- Develop a comprehensive approach – to harness the full advantages of augmented intelligence for the enterprise, you must connect people, processes, data, and technology across the organization
- Build a strong foundation for innovation – actions like migrating your data and analytics to the cloud can help you increase agility, flexibility, and scalability
- Seek industry and functional expertise – experience matters, so take the time to find industry and technology partners that can help bring your vision to life
- Embrace a data-driven culture – data is an integral part of every function, and all employees must have the tools to unlock its power
- Purpose leads to profits – to build a resilient organization, focus on long-term value creation for all
Visit our data and analytics solutions page
To remain relevant in the digital age, businesses must continue to evolve. With augmented decision-making at the core, enterprise leaders can ensure their organizations move faster than market demand, stay a step ahead of the competition, and, ultimately, create memorable experiences that keep employees, customers, and investors coming back for more.