- Blog
How financial institutions can fight rising scam fraud and financial crime
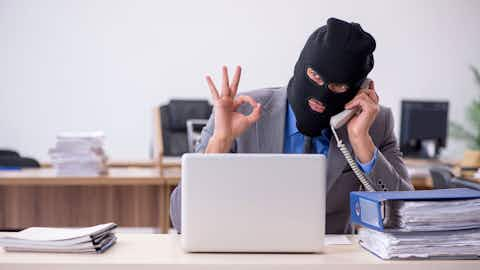
Break down internal silos to fight rising fraud with AI, cloud, and analytics
We expect fraud in online, mobile, and buy-now-pay-later channels to rise as the number of transactions on these channels continues to increase. Scam fraud and authorized push payments fraud will also continue to increase due to social engineering schemes. Since last year, UK regulators have been advising banks to reimburse customers tricked by scams. Regulators in other geographies are likely to follow suit, requiring banks to swallow more of the loss.
Fortunately, cloud, analytics, and AI-based security solutions will help drive fraud prevention and detection. According to a Fortune Business Insights study from March 2022, this will result in a 22.8% growth in the global fraud detection and prevention market over the next seven years, with the market expanding from $30.65 billion in 2022 to $129.17 billion in 2029.
Reduce financial crime more effectively with entity-centric monitoring
Companies will increasingly focus on efficiency and cost reduction. Not surprisingly, then, the roughly $274 billion spent globally on financial crime compliance per year will be a key target for cost-cutting. So, we expect to see a seismic shift in the sector's focus from event-driven to entity-centric monitoring, which is best served by advanced digital technologies.
With event-driven monitoring, a customer's legitimate transaction (the 'event') is often flagged as suspicious, resulting in a 'false positive'. By some estimates, around 95% of system-generated alerts are false positives. However, banks will turn to artificial intelligence to replicate the complex reviews of these false positive alerts that humans had previously performed. This will result in the same regulatorily defensible case files produced at greater speed and reduced cost while freeing up analysts and human investigators to do more value-added work.
An entity-centric approach means presenting data from the perspective of entities and their relationships, rather than individual events. So, it also looks at many more factors than previous methods. This type of contextual assessment results in better detection, investigations, and outcomes. Contextual understanding boils down to having the correct data and intelligence. So, the large-scale use of cloud computing will be essential for this approach.
Stay tuned for our next two predictions in this series.