- Blog
Five building blocks that turn insights into action
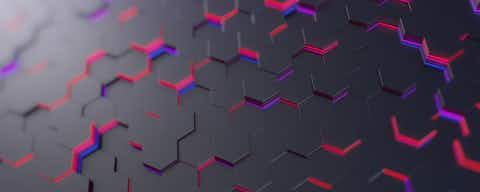
Is your financial planning and analysis (FP&A) team investing its time well? A key activity for FP&A is to support business decision-making and recommend actions. But most functions spend too much time gathering and analyzing data to uncover insights, and not enough turning these insights into action.
We see this as two loops: data to insights, and insights to action. And the first currently demands too much effort and time.
Some of the world's most successful companies give credit to their ability to turn insights into action at the right time for their growth. Here's what a number of CEOs have said about their focus on data, insights and action in their annual reports:
GE: We plan to lead in the next wave of industrial productivity. Last year, we invested about $4 billion to build out our analytics software and machine learning capability
BP: Modernizing the whole group: we will be deploying advanced technologies such as robotics and big data analytics to improve and simplify our processes
To harness the full potential of both loops, we have identified five building blocks that lead to effective decision making.
Figure 1: The five building blocks for effective decision making
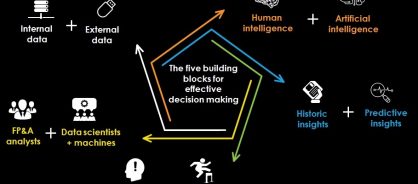
Block 1: Internal data + external data
In today's economy, data is more valuable than oil. While there is no shortage of data, it's the companies that manage it well that create competitive advantage. As is often reported, 90% of the data in the world has been created in the past two years, at 2.5 quintillion bytes of data a day. And 80% of it is unstructured, in emails, social likes, call scripts, news, or audio, for example. The challenge lies in how to harness it.
Executives have always used data to support their viewpoints. What they need now is the ability to use data to generate insights, not merely support them. They need a transparent and robust mechanism to get to the right data faster, if not in real time.
Technology developments in robotic automation, data processing and storage, in-memory computing and big data, mean we're no longer focused on how we manage data – the master data structure, data dimensions, and hierarchies. We must now focus on what data to use, how to use and apply it in an industry and business context.
Block 2: artificial intelligence + human intelligence = super intelligence
As automation and technology continue to advance, so does talk of machines replacing humans. But history has taught us that people who can work effectively with machines are more likely to succeed.
An AI robot recently took the entrance exam for Tokyo University: a mix of math, reasoning, essay writing, and multiple choice questions. Despite access to search engines and source content, it failed.
AI can only augment people's ability to rapidly read, analyze and interpret data. Here are a couple of examples:
- AI can generate automated reports based on internal and external data, but people add the context and the relevance of company culture and strategy, for example
- AI studies data to identify patterns and adjustments based on structured algorithms, trends and even factoids. People add reasoning to these results to make more nuanced choices
Block 3. Historical + predictive insights
Mobility and data analytics tools like Tableau and Qlik Sense enable real-time reporting and what-if analysis through data visualization. And machine learning and natural language generation allow organizations to automate commentary writing and insight generation.
But trying to identify trends or provide descriptive analysis is next to pointless in an ever-changing environment. This is why predictive models and insights are paramount.
A global consumer goods firm offers a good example. By combining a predictive model and sensor-enabled refrigerator, it has halved its out-of-stock inventory.
Block 4: Insights + action
The question for finance leaders is not whether they're getting the right reports but if they're asking the right questions. Next they need to measure the actions taken against the insights generated so they can improve insights.
When a consumer goods firm used zero-based budgeting on its overhead costs its FP&A teams had to take immediate action on cost. We helped implement an app with better visualization and analytics for different expenses and ensure the right analytics reach the right cost owners. It measures each action too.
Block 5: FP and A dream team = analysts + data scientists + artificial intelligence
There are two key skills-related themes when predicting the future of FP&A:
1.1. Evolving roles: As machines do the logical, fact-based left-brain activities, we need to redefine the skills needed in FP&A to deliver the intuitive, subjective, and variable right-brain activities. Technology has automated data gathering, rationalization, visualization, and analytics. But all the data, logical relationships, and algorithms must be well trained, contextualized and reasoned without bias by people
1.2. The gig economy: As more people, especially millennials, now prefer short-term contracts, what impact will this have on FP&A? How can right-brain activities be shared with the talent available?
Organizations must determine how best to respond to evolving roles and a changing economy.
The future of FP&A lies in combining advanced technology with evolved FP&A skills and business partnering. The pace of change and complexity in business continues to increase at speed. To keep up, FP&A must evolve while challenging its drivers, data sources, analytic algorithms, and skill sets.
Our five building blocks offer the foundations for change so you can harness the full potential of the data-to-insight and insight-to-action loops.
Read more about the future of FP&A: Data, digital, and skills: the new mantra for enterprise performance management.