- Blog
Driving growth through natural language text
The market for natural language understanding is set to take off. Here's what you need to know
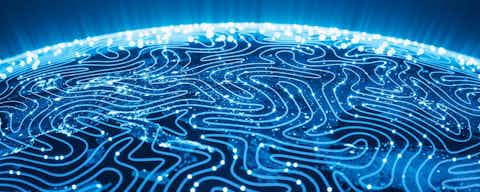
Over the last 25 years, the internet has created an explosion of text and data. Consumers today are doing far more than merely buying and selling goods online. They're also soaking up and generating a great deal of valuable data. Because most of this data is text-based and complex, deriving insights from it has been hard.
That's changing, as digital technologies evolve. Solutions that handle unstructured text can be a real game-changer. For instance, by picking up nuances in language, they can make a big difference when it comes to getting the right Google search result. They can also help us interpret social-media chatter to better understand human behavior.
Natural language processing (NLP), the means by which a computer absorbs, dissects, and analyzes text or speech, turns this data into encoded, structured information, then proposes actions based on the output in everyday language. Natural language understanding (NLU), a subset of NLP, parses unstructured inputs, then structures it in a way that both the machine and humans can understand and act upon.
NLU has captured attention in some industries by answering complex business problems. For example, it can help identify adverse events in drug safety reports, improve patient care by mining medical data, and retrieve information from lengthy contract documents. Yet, for the most part, industry hasn't exploited it for insights or invested in analyzing the rich motherlode of unstructured textual data originating from multiple online sources.
A learning curve is in order
For NLU to meet its full potential – and to best position it in the marketplace – both providers and industry at large need to better understand the technology. Should we categorize NLU, for example, as a machine-learning, technology-centric, information-mining product? Or is it an extension of content and knowledge management systems that classify, store, and deliver documents? And does it contribute most when integrated into operations such as customer relationship management systems? In fact, the answer is yes to all of the above, but it's largely up to data scientists to provide the right NLU approach to each business problem.
The nuts and bolts of NLU
Textual data analytics provides deeper insights about consumer behavior and experiences. It can also get to the root cause of problems customers encounter when interacting with a company. But harnessing the power of text to derive these insights depends on using not just the traditional NLU process (Figure 1), but the right NLU approach and methodology.
Today, there are more NLP and NLU tools than ever, as language engineers build new applications with a range of algorithms and processes.
Figure 1: The traditional NLU pipeline
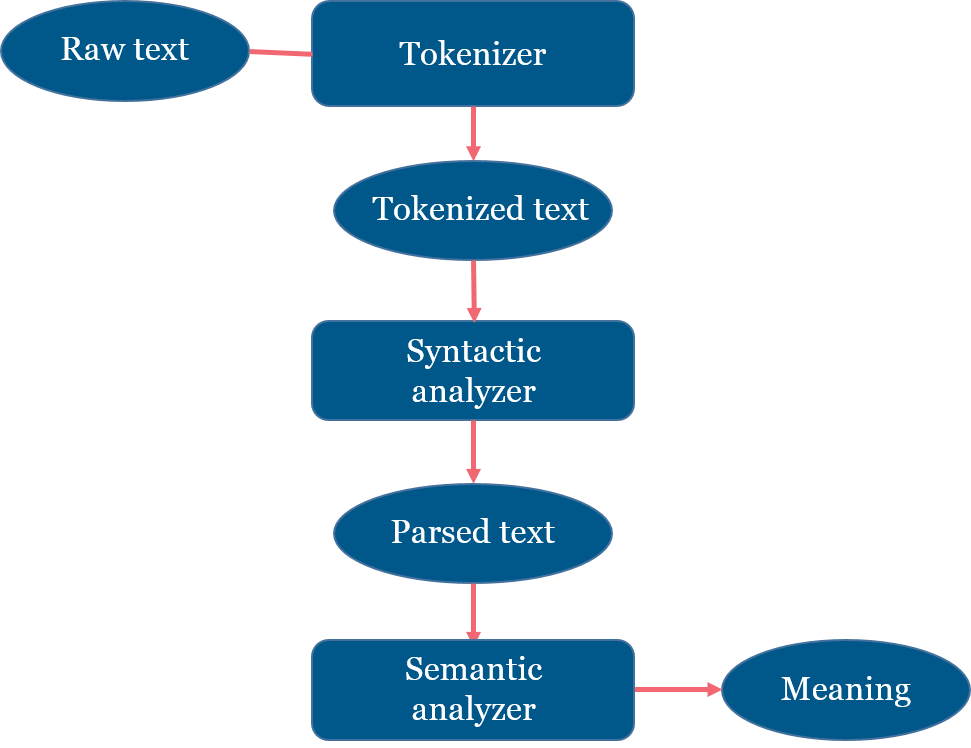
NLU's limitations and challenges
At the same time, adapting applications, tools, and algorithms to build a robust pipeline across industries and verticals can be challenging for the following reasons:
- NLU applications don't always provide the context of the text in content
- Because there are many different ways of saying things, tools need to cope with enormous variation
- The same piece of text can have many distinct interpretations
- Diverse linguistic styles, such as formal/informal, technical/nontechnical, and social-media formats are a challenge
- Text is often badly formed or fragmented
- As language use evolves, many new terms and new meanings of existing terms develop
- Language use is highly creative, so literal interpretations sometimes fail
- Understanding a language requires knowledge of the world – a problem artificial intelligence (AI) may ultimately address
Applications across industries
But even with these challenges, there are many circumstances where NLU is adding real value. Examples include:
- Using AI and NLU in healthcare to improve the patient experience through auto-categorization and sentiment analysis
- Applying NLU and machine learning to mine, extract, and analyze unstructured contracts as a means of identifying revenue leakages and losses
- Using NLU and machine learning to mine, extract, and analyze unstructured web chats to identify inflection points and suggest ideal responses for improved customer experience
- Using NLU to identify potential channel or experience breakages through the analysis of customer feedback and interactions
Developing a go-to-market strategy
The NLU market is new, fluid, and ready to take off. To make the most of the opportunity, data scientists must assess and validate NLU developments frequently, refining their packaging, positioning, and approaches accordingly. And those selling the product must understand what it is, know their competitors, and consider alliances and business synergies that could exploit this technology.
Doing so could prove truly worthwhile. We're producing and consuming more and more text every minute. So the room for growth and impact in the field is huge.