- Point of view
Decision mining: The next frontier in supply chain operations
How to extract more value from advanced planning systems using decision mining
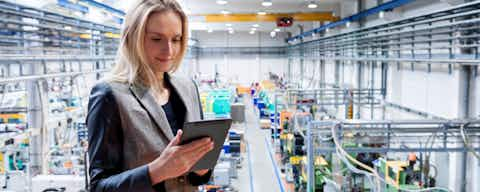
Most organizations use an advanced planning system (APS). The question is, does your business under-use its APS?
Implemented in conjunction with an enterprise resource planning (ERP) system, the APS promised to revolutionize supply chain planning by delivering automated recommendations based on supply chain management best practices. This, in turn, would enable planners to focus on higher-value tasks and actions.
Unfortunately, in many organizations, APS performance falls short of its promise – not because of shortcomings with the platform or its capabilities, but because it's not used to its full potential.
Though APS platforms host an impressive array of features, some planners simply do not trust system recommendations, which leads to system overrides and manual processing. This not only creates inefficiencies but can also negatively impact outcomes.
In this way, the APS' failure to meet expectations isn't a matter of under-delivery so much as under-utilization – and this issue is far more common than you might think.
Seizing the full value from the APS through decision mining
For the sake of our argument, let's assume that there's nothing wrong with the underlying APS – that the software selection, configuration, implementation, and training were all completed properly. If this is the case, the system algorithms will deliver the optimal recommendations in almost every instance. In other words, decision overrides should be used sparingly, if at all.
And yet, in many organizations, manual decisioning is a regular occurrence. This means that planners are not allowing the APS to deliver on its promise.
But to change the way the business uses the APS, it's necessary to prove its value by demonstrating how overrides lead to suboptimal results compared to the recommendations produced by the APS. Perhaps more importantly, the business should also be able to correlate optimal outcomes with certain steps, techniques, or attributes within the decision-making process in order to replicate them in the future.
Take a copy for yourself
But how does an organization determine whether it's under-using the APS and how this is affecting the business? Our answer is simple: by using decision mining.
How to extract more value from your APS
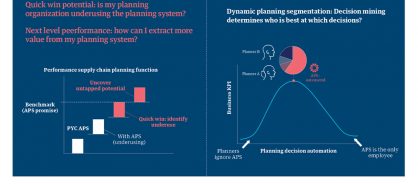
Three steps to better, smarter supply chain operations with decision mining
Decision mining is the systematic capture and analysis of supply chain decisions for improved efficiency, better outcomes, and an enhanced customer experience.
Our decision mining framework follows three main steps:
- Capture decision data within the context of the planning process, such as sales and operations (S&OP) planning or integrated business planning. During this phase, data will be organized according to its process step, time stamp, and sequence. It will also be compared to the optimal path identified by the APS
- Actual planning process data is matched and analyzed against the performance projected by the APS. This data is also analyzed according to a wealth of attributes, such as product groups, vendors, geographies, the presence/absence of process steps, planners, and segments, to determine what factors or combination of factors drive optimal decision-making
- Recommendations produced by the APS. These may include:
- Planning segmentation: which planner should plan which part during which season
- A tailored training program: planning practices to add maximum value to the process
- Improvement initiatives: which segment, vendor, geography, or other category is generating a disproportionate cost to the organization
Through this three-step process, decision mining identifies which features within the APS are under-used and recommends areas for improvement.
Powering the decision mining engine with process mining and machine learning
Our decision mining methodology relies on the use of two specific capabilities: process mining and machine learning.
Process mining
Process mining optimizes business processes by analyzing event log data from the ERP in order to help organizations visualize how processes within the supply chain actually run, as opposed to how they ought to. Process mining is necessary to understand decisions and actions in the context of actual business processes.
Machine learning
Machine learning recognizes the patterns that result in value-adding or value-diminishing decisions. Given the limitations of human processing power, computer-generated algorithms are far more efficient (and effective) at identifying these patterns. This in turn allows planners to focus on higher-value tasks, as opposed to time-consuming and complex analysis.
Decision mining leverages process mining and machine learning to determine which APS features are under-used and identify areas of improvement.
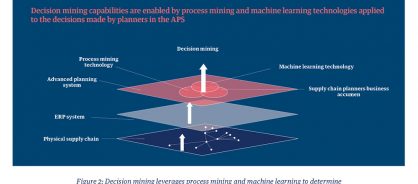
Applying the power of decision mining to your business
Business operations are continuously evolving as new technologies create new capabilities. First came ERP (which revolutionized the planning function) followed by the introduction of the APS, which streamlined supply chain operations through automation. Today, the next logical step is to scientifically improve decision-making using decision mining.
If your supply chain has an APS in place, it's time to make sure your planning function is using it to its fullest potential. It may just be your organization's best decision yet.
This point of view was authored by Luis Dominguez, supply chain solutions, Genpact.