- Blog
AI enhances patient, clinician experience
Take coding and billing to the next level
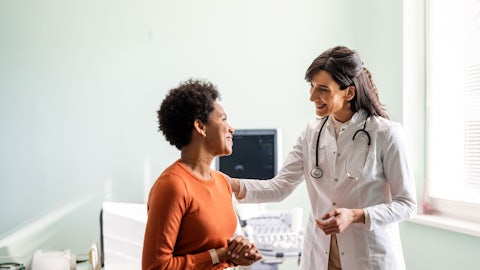
Healthcare news site Healio recently published my views on how healthcare providers can use AI to take over coding and billing tasks, giving clinicians more time to focus on their patients. Here's a short extract and a link to the full piece.
Medical coding has historically been a manual and disjointed process, with an office manager, coding specialist, or clinician reviewing the data after a patient visit and coding to the best of their abilities.
As an input, clinicians are often required to update the patient chart in the electronic medical record (EMR), usually during the visit. The completion of these fields is so important, it drives the flow of every patient conversation, interrupting a natural, fully attentive exchange.
Now, as the healthcare industry looks for ways to ease clinician burnout, improve the patient experience, and gain efficiencies, it's time for coding and billing to evolve again – this time with an assist from artificial intelligence (AI). And that includes the newest technology on everyone's minds, generative AI.
AI makes it possible for coding to happen in real time, starting during the patient visit, and not after. Using technology to rethink how and when we collect the inputs for coding can have an immediate and positive impact on the administrative workload and patient and clinician experience.
AI: A technology for today
There's a lot of talk about how healthcare will use AI in the future. But for coding and billing, we don't have to wait. The technology is available, accessible, and usable today.
With AI, data collection for coding begins as soon as your conversation with the patient starts in the care room.
Read the full article on Healio to learn how AI and generative AI are reducing the burden on clinicians so they can make care more human-centric. And get the four steps to AI success, too.