- Video
Women in supply chain
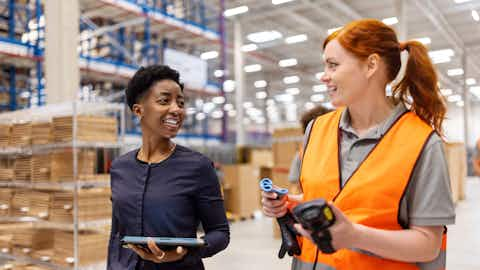
A more equitable future – and a competitive advantage
The battle for supply chain talent is fierce. The Great Resignation and the impact of COVID-19 on working women have led to huge numbers of unfilled vacancies at a time when supply chains need experienced employees to lead them through continued volatility. Improving the recruitment, retention, and development of female talent in supply chains is not just the right thing to do, but it is also a business imperative.
This series of conversations between Genpact and some of the world's leading brands covers topics from supply chain recruitment to work-life balance and the power of mentors to increase female representation at all levels of the supply chain. They offer practical steps for personal and professional growth and highlight the opportunities available to women.
The magic of mentors
Helping women reach the top
How The Coca-Cola Company and Genpact are supporting female supply chain talent to climb the career ladder.
The power of mentors and networking
How former bosses and peers hold the key to career success.
Knowing what I know now
Advice for younger supply chain talent.
The balancing act
Setting the right expectations
How Genpact and Danone encourage employees to balance flexibility and accountability in a virtual environment.
Building an ecosystem of allies
You don't have to do it alone. A trusted group of advisors can help navigate your career choices better.
Bringing your passions to work
Explore passions inside and outside work to enjoy a fulfilling personal and professional life.
Join Genpact's supply chain team
Genpact's supply chain team is always on the hunt for new talent. Visit our careers page to search current opportunities.
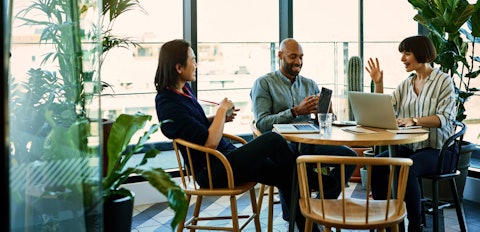
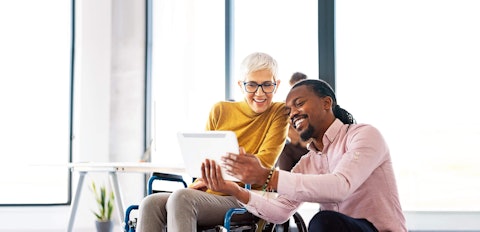