- Blog
How high tech companies can grow customer profitability with machine learning
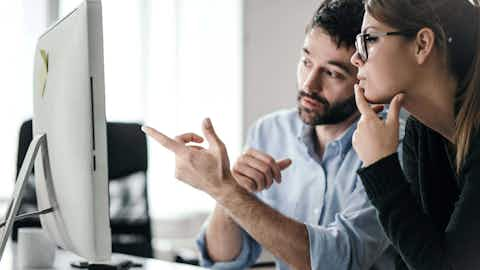
In today's uncertain economic environment, one thing you can still count on is that when your customers do better, your company will thrive. One way to achieve this is by improving operational performance, but there are hundreds of business metrics to consider. But what are the best metrics for high-tech OEM and services companies to focus on in the current environment?
Certainly, overall corporate profitability is critical, but another important measure to consider is customer experience-to-profitability view. This approach looks at your account base on both a revenue and cost basis through the lens of customer experience. This may seem obvious, but in my experience, I've found that many companies don't do this at all or don't do it well, either because of challenges gathering the required data from multiple functions, process definition difficulties, or issues with master data customer definition.
How to increase customer profitability with machine learning
So, what is the best way to improve customer profitability? The simple answer is to make your customers more successful.
For a gross average, you could take your total revenue and divide it by the number of customers, giving the total margin per customer. But pretty much every business has some kind of 80/20 rule by which roughly 20% of customers provide 80% of revenue. Or at least where different customers contribute very differently to the bottom line. High-touch customers that need a lot of customer success managers and hand-holding will naturally have a higher cost to serve. But sometimes, customers that don't contribute much revenue also cost a lot to manage. Sometimes, higher-revenue customers can have lower overhead costs. You need to have a clear picture of what your best customers look like.
Here's where machine learning (ML) and pattern recognition come in. This analyzes the business interactions that have led to better-performing customer profitability segments, mapping the customer journey and profitability at an account level. There will be a distribution that can be analyzed based on sales data, renewals, customer support, and service yield patterns in customer responses, behavior, and outcomes.
Better data will give better signals that yield more intelligence and better results. But even basic data will produce useful insights. The data can come from existing sources such as customer experience or health, process mining, Net Promoter Scores, and interaction quality metrics. The key is to then combine and aggregate the sources together correctly.
ML models find patterns in the customer journey and map these to profitability segments using customer revenue and cost to serve (see figure 1). By studying the map, your business can learn which customer journey patterns lead to better customer profitability performance and then enhance the successful outcomes that optimize customer profitability.
Figure 1: How customer experience maps to profitability
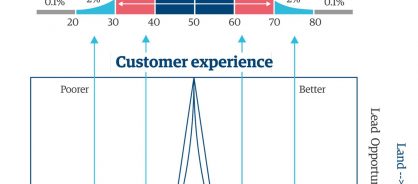
Unfortunately, you won't find one perfect piece of software to plug-and-play customer profitability, but Genpact's modular suite of analytics and AI tools can deliver results in less than three months, giving you a repeatable and reliable customer profitability measurement system going forward. If you're looking to simultaneously ensure customer success and enhance customer profitability in this uncertain economic environment, machine learning is the way forward.