- Case study
Transforming finance for a global insurer: A new operating model with the tools and mindset for change
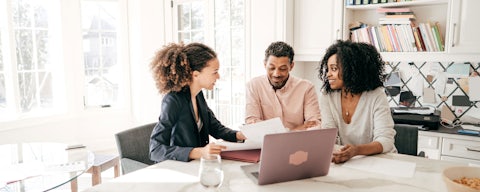
Who we worked with
One of the largest global providers of insurance products, covering property, casualty, accident, and health as well as reinsurance and life insurance products in multiple countries and territories.
How we helped
Using a blueprinting approach, we worked closely with the insurer to transform from within. We designed and deployed an advanced finance target operating model (TOM) to simplify and standardize global processes and accelerate transformation.
What the company needed
Faced with complex and fragmented processes that were stifling growth and transformation, this insurer needed a simpler, scalable, more efficient finance operating model to speed up change and enhance competitiveness.
What the company got
Standardized finance processes supported by best-in-class automation and digital solutions. The company is more agile, has tighter governance and controls, and now embraces an end-to-end transformation mindset. Run rate, overall efficiency, and user experience are up, and operating costs are down across financial planning and analysis, accounts receivable, treasury, and reconciliations processes.
Take a copy for yourself
Challenge
Gain buy-in and simplify to transform
Nonstandardized processes across global finance operations were hampering growth and adding costs for this insurer. Problems included intensive manual reporting processes, inconsistent guidelines, no global accounts receivable tool, and different reconciliation processes across segments of business.
It already knew it had to standardize processes to achieve the efficiencies, speed of growth, and cost savings it desired and had embarked on a global transformation program. But with that program focused on broader technology and tool implementation, there was an opportunity to augment this with a detailed roadmap to guide it to completion. It selected Genpact, its partner of more than 10 years, to evaluate its finance operations for accelerated process transformation, implement more specific digital and IT solutions, and drive an end-to-end transformation program.
Visit our insurance solutions page
Solution
Detailed roadmaps to navigate change
To identify the biggest opportunities for transformation that would meet the different objectives of the various parts of the business involved, we began with an in-depth review of finance operations and a due diligence review of processes across business segments and geographies for an insider's view of the business. We found two key problems:
- Although the multiple initiatives within the transformation program all had individual owners, it was a challenge to align global leads and resources to drive wider change across geographies and business segments
- Initiative owners in the transformation program had focused on system implementation rather than end-to-end transformation
We suggested several solutions to overcome these barriers to change:
- By showcasing global scenarios and best practices across the industry, we could act as a change agent to encourage resource matching and help initiative owners envisage the full transformation journey, break down silos, and fix fragmented processes
- By conducting thorough due diligence, we could demonstrate savings targets backed by facts and supported with a future roadmap
- We suggested global blueprinting, mapping each region in detail to identify every process and bring consistency and standardization across its financial planning and analysis processes
- We combined our process and insurance skills to design the missing components of the existing TOM
Our program of work with the client is underway:
- We created a blueprint TOM for treasury operations in North America
- For accounts receivable and global collections, we're accelerating the TOM journey and supporting to-be state process standardization across regions
- We're supporting reconciliation platform implementation in three regions for balance sheet reconciliations
- Financial planning and accounting – we're supporting the design of a future- state TOM and self-service reporting with a team of data scientists and digital technology experts
Impact
Faster end-to-end transformation
Genpact has accelerated finance transformation with scalable, strategic solutions and standardized global processes that have delivered greater governance and visibility across the finance function. We've also achieved operational efficiencies by consolidating operations into a global shared services model.
As a result of the outcomes so far, the client has asked us to help build dedicated teams to automate reporting and treasury operations with reconciliation platform implementation across geographies and business units as well as global TOM implementation for collections.