- Case study
Turning an energy business into a predictive-maintenance powerhouse
How to keep crucial turbines running with more accurate forecasting
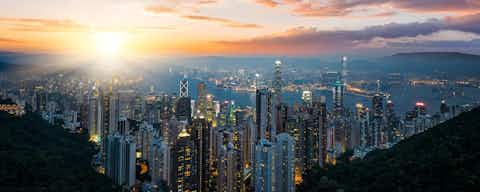
Who we worked with
A leading original equipment manufacturer (OEM) that provides turbines, spare parts, and services to utility companies worldwide
How we helped
We used machine learning (ML), artificial intelligence (AI), and advanced analytics to predict turbine-equipment issues and maintenance needs more accurately
What the company needed
To help its utility-company clients increase turbine availability using predictive maintenance
What the company got
- Higher customer satisfaction from improved turbine up-time
- Data-driven insights for smarter business decisions
- More effective use of resources
Challenge
Increase turbine up-time by making maintenance more predictable and reliable
This global energy leader provides turbine engines to large-scale utility companies around the world.
Typically, half of all parts failures occur unexpectedly at utilities' operations. And when problems arise, power generation suffers while companies wait for replacement pieces and field-worker repairs. Meanwhile, if there are turbine stoppages, utility companies may experience power outages that impact their commitments to their own customers.
Part of the problem was that until now, maintenance forecasting had been limited to using simple extrapolation models. To be effective, these models needed to interpret a diverse array of structured and unstructured data. But that was not an easy task.
But it's changing.
The energy firm knew it had to find a better, more comprehensive way to analyze its data. Its operational and inspection records, machine specifications, and equipment-imaging devices held a wealth of intelligence. Using such rich, science-driven information was the only way the company could boost customer satisfaction by accurately predicting parts failures to keep the turbines running. This data could even reveal new business opportunities, while also helping the company allocate maintenance resources at the right time and level of detail.
Solution
Digitally powered technology that improves prediction capabilities
Genpact had already been working with businesses to improve maintenance predictability and created our unique Smart Event Forecasting solution. The technology combines process knowledge, digital technology, and predictive analytics to sharply improve the accuracy of parts-breakdown forecasts.
Smart Event Forecasting combines natural language processing (NLP), ML, neural networks, and advanced visualization to interpret a range of structured and unstructured data. After it deciphers all the factors influencing potential parts failure and machine breakdowns, it delivers predictive maintenance scenarios to decision makers. It also uses accessible visualization with charts and images so they can also understand the information quickly and easily.
The solution interprets and integrates data, such as inspection reports, operational information, specifications, and equipment images, and uses the following key components:
- Text mining: Our NLP model interprets unstructured inspection reports to determine the current state of equipment and classify opportunities for anticipating events
- Machine learning: Our model integrates process data with historical operating intelligence to predict future parts failure. The system then selects the best fit from more than 50 possible distributions
- Deep learning: The solution analyzes equipment images using a deep-learning neural network to classify damaged parts and identify the scope of potential interventions
- Advanced visualization: The results from these tools are then integrated and presented to operators through a dashboard using advanced visualization to identify potential actions. Key elements include:
- Classification of maintenance concerns into those that need action, continued monitoring, or no action. It also identifies processes with single or multiple concerns
- Image displays of machine parts showing wear or damage
- Calculations of future event probabilities based on equipment operating hours
The system incorporates decades of reports and generates best-fit probability distributions based on techniques that Genpact has developed with leading universities. This makes the solution considerably more accurate than similar tools. In fact, it predicts future events with more than 90% accuracy, compared to about 80% using traditional modeling techniques.
Smart Event Forecasting can be scaled and used in any manufacturing process. But its remarkable versatility extends beyond manufacturing. In fact, it's effective in almost any operation, such as accounting or IT, where the parameters driving failure can be identified and have relevant data available. Its potential applications are vast.
Impact
Cutting downtime. Increasing customer satisfaction
By predicting events in manufacturing processes more accurately, our client has:
- Delighted its customers by reducing turbine downtime
- Cut the penalties that its utility-company clients must pay their customers when turbines fail
- Kept the lights on for end users around the world
- Made smarter business decisions using data-driven insights
- Generated greater efficiency from resources and maintenance planning
- Saved $3 million in field-service costs in less than one year
This global manufacturer is extending the benefits of Smart Event Forecasting by rolling it out at many of its production sites. It also has plans to extend these sharper insights and efficiencies throughout its operations.