- Case study
Future-proofing compliance with cloud
How a leading online broker boosted compliance with riskCanvas, a cloud-native financial crime software suite
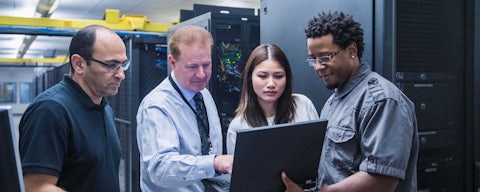
Who we worked with:
A leading US online broker that offers an electronic platform for stock trading, investing, and retirement planning. The broker has more than 1 million accounts and assets under management exceeding $1 trillion.
What the company needed:
A much clearer view of – and control over – every aspect of compliance for greater efficiency and improved business insights to avoid costly fines and reputational damage.
How we helped:
Implemented riskCanvasTM, our financial crime software suite, to enable the firm to detect, investigate, and prevent a variety of threats.
What the company got:
- Merged 60+ workflows across multiple systems into a single interface
- The ability to track and monitor key performance indicators more precisely and develop dynamic dashboards that answer business-critical questions
- A better employee experience for multiple teams and workforces
- The flexibility to more easily meet new and tougher due diligence requirements
Challenge
Staying one step ahead of compliance regulations
This pioneer in online investing has a long-standing reputation for making trading intuitive and accessible. But the firm was finding it hard to keep pace with fast-changing compliance regulations.
Unfortunately, disconnected teams and processes made compliance complex. Employees were even manually managing some of the compliance processes. As a result, quality standards were dangerously inconsistent, data that would have been valuable for producing business insights fell through the cracks, and process inefficiencies caused costs to climb.
Worse still, these challenges put the firm at considerable risk of compliance failure – which could lead to multimillion-dollar fines and reputational damage.
Take a copy for yourself
The solution
A cloud-based approach to reduce risk
The company chose riskCanvas, Genpact's financial crime software suite, to address its compliance concerns for three reasons:
- They could configure it to support bespoke workflows.
- They could easily integrate it with their existing data.
- It offered scalability and flexibility – it could keep pace with a dynamic environment while optimizing usage within cost constraints.
When the company brought Genpact in, our first priority was to fully analyze the pain points and weaknesses in the company's existing compliance framework. We held in-depth discovery sessions with its chief risk officer and every other stakeholder who touched the compliance process. We then brought in industry-leading compliance experts with government and frontline experience to work with the firm's compliance team. Together, we identified solutions for every problem across process, technology, culture, and organizational structure.
Next, we developed a proof of concept using riskCanvas. This showed how the application could overhaul workflows and integrate processes for greater efficiency. Plus, riskCanvas' flexible architecture integrated different datasets and built configurable workflows tailored to the company's needs impressed stakeholders. When they saw how well the application worked with Amazon Web Services (AWS) cloud capabilities, it sold them. AWS supports the flexibility and scalability the firm needs for a future-proof compliance solution.
The impact
Security, insights, and savings
The solution helped the firm to:
- Merge 60+ workflows across multiple systems into a single interface providing easy access to clean, valuable, and shareable data
- Track and monitor key performance indicators more precisely because riskCanvas stores a variety of data to make business intelligence and analytics much easier
- Develop dynamic dashboards that answer business-critical questions around customer risk exposure, cross-business risk posture, and team efficiency
- Improve the employee experience for multiple teams and workforces
- Retire a costly legacy trading account reporting tool and more easily meet new and tougher due diligence requirements
- Reduce the need for expensive licenses and support tools
Today, using riskCanvas' risk scoring system, the firm is confident that it not only meets but exceeds due diligence requirements. This has virtually eliminated the risk of huge fines and reputational damage.
What's more, the migration to AWS has saved the firm millions of dollars. Computers, which now run overnight uploading and processing materials, are far more cost-effective. Plus, the company is saving millions in licensing fees, support tools, person-hours, and managed service fees.
But it doesn't stop there. The brokerage expects to see year-over-year improvements as the system implements new architectural changes, onboards new business processes, and updates new federal requirements in real time.