- Case study
Blending technology and AI for specialty underwriting
How a reinsurer makes data-driven decisions across the globe
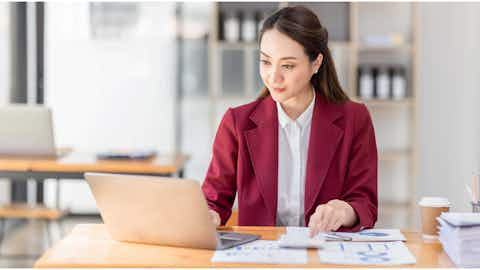
Who we worked with
One of the top three global reinsurers
What the company needed
- Significantly reduce the response time to ceding carriers – the insurers that pass all or some of their risks to another insurer
- Evaluate underwriting risks at speed
- A modernized digital, data, and cloud infrastructure to lay the foundation for artificial intelligence (AI) and machine learning (ML) technologies
How we helped
- Developed a centralized view of data from multiple sources
- Built a predictive risk evaluation solution associated with a ceding carrier's inquiry, powered by AI and ML technologies
- Enabled underwriters to add risk-specific metrics to individual cases, improving decision-making
What the company got
- A unified view of relevant historical risk data to aid with data-driven decisions
- Improved risk selection to determine adequate coverage and premiums, resulting in new revenue
- The potential to increase top-line revenue by 12–15% annually with improved response time thanks to ML models
- Improved underwriter efficiency and productivity
- A faster response time and better customer experience for ceding carriers
Challenge
Speed up underwriting response times or lose business
As an expert in risk management, this reinsurer knows that a good underwriting process calls for data-driven insights. And though business was booming, its processes were more manual than modern.
Underwriters had to review vast amounts of data from multiple sources to make decisions about risk and pricing. As a result, it would often take over a week to respond to ceding carriers.
Worse still, new insurtech companies entered the market. Though they lacked experience, they used digital tools like ML to rapidly perform qualitative risk analysis and quantitative risk analysis – responding to ceding carrier inquiries in hours instead of days.
The reinsurer set an ambitious goal to respond to more than a third of requests from ceding carriers within two hours. This drastic reduction in turnaround time would lead to higher market share and top-line growth.
But to achieve this, the reinsurer needed to address a significant problem: revamping its IT infrastructure. After years of rapid growth, the company's infrastructure had become disorganized and unconnected and required more governance. In addition, continuous integration, and data delivery – a prerequisite to ML – would be impossible with the company's siloed systems.
Solution
AI insights fuel accelerated underwriting
Determined to revamp its operations, the reinsurer's leadership team started a complete digital transformation of systems and processes. The focus was to explore how advanced analytics, data science, and machine learning could support faster and more informed decisions.
Genpact was the chosen partner for this initiative because of our data, analytics, and digital expertise, and our track record of reimagining thousands of operations in the insurance industry.
So, we jumped into action. First, we developed a blueprint to get to a cloud-based infrastructure that would support the data needed for ML. We then connected data across multiple systems into one data pipeline. This approach allowed us to design two AI-driven risk prediction models based on the company's historical risk data:
- Model 1 predicts the likelihood of risk falling within the reinsurer's underwriting remit
- Model 2 predicts the likelihood that these risks will remain with the ceding carrier
The solution was developed on Amazon Web Services (AWS) provided eco-system within the reinsurer's infrastructure. Our AI experts then developed a data pipeline, on AWS, integrating new and varied data sources. This approach enhanced the risk prediction models with diverse datasets to continuously improve their accuracy. Plus, the system saves all the different versions of the models so the reinsurer has a repository of ML models they can test or deploy at any time in the future.
Finally, we added the ability for underwriters to add case-specific information without affecting underlying algorithms. Viewing the output of the AI models through a user-friendly dashboard, underwriters can input their feedback, which aids in continuous machine learning and in refining predictive outcomes.
What's more, they have a complete and transparent view of the data that feeds these models to build trust in the insights the ML models provide, which, in turn, boosts user adoption.
Impact
Data-driven decisions across the globe
Today, people, processes, and data work hand in hand. It's a perfect example of augmented intelligence – where humans and machines work together to learn from each other and improve continually.
With data at the core, underwriters can confidently make rapid decisions – and respond to ceding carrier inquiries within two hours.
The new ML models also help the reinsurer:
- Reduce decision time: The ML models take just 14 seconds to produce results for 200 entries. This development enables underwriters to respond to 30–35% of cases more quickly
- Improve risk selection: ML covers both routine and complex risks, generating data-driven insights to guide decision-making every time
- Enhance the customer experience: Carriers want to work with reinsurers who respond quickly to their needs. By drastically reducing turnaround time, the reinsurer can attract and retain more business
- Boost underwriter productivity and job satisfaction: With ML models automatically determining which opportunities meet underwriting appetite and high placement likelihood, underwriters can refocus on higher-value work
By combining deep underwriting expertise with the best machine learning technology available, this global reinsurer is prepared for new emerging risks and new sources of competition.